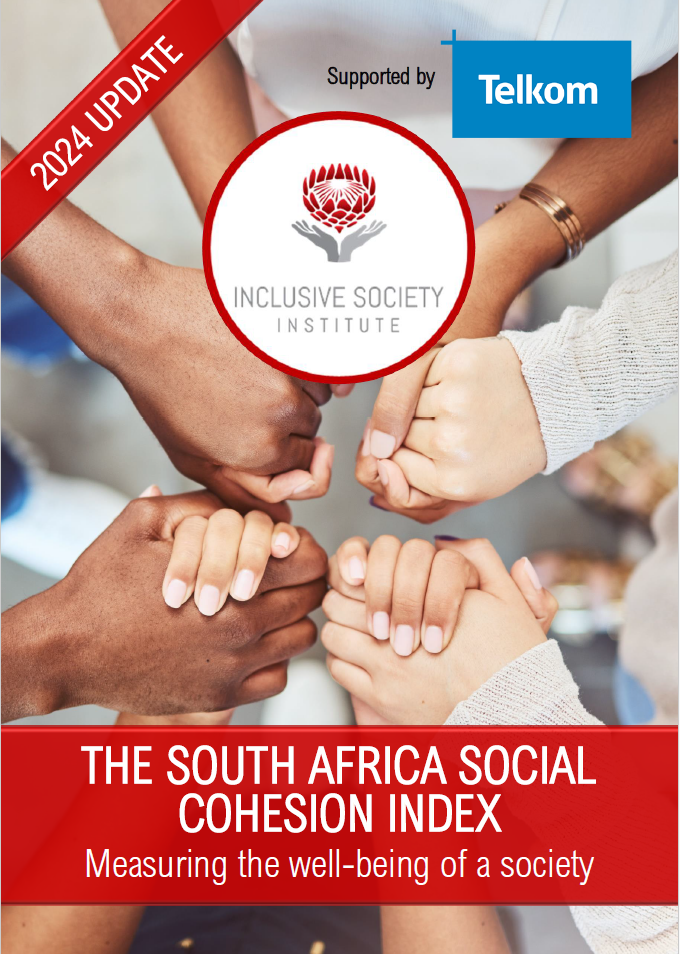
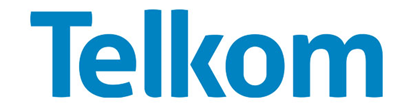
This report has been enabled
through the generous support of
Telkom
Copyright © 2025
Inclusive Society Institute
PO Box 12609
Mill Street
Cape Town, 8010
South Africa
235-515 NPO
All rights reserved. No part of this publication may be reproduced or transmitted in any form or by any means without the permission in writing from the Inclusive Society Institute
DISCLAIMER
Views expressed in this report do not necessarily represent the views of the Inclusive Society Institute or its Board or Council members.
February 2025
Author: Georgi Dragolov and Klaus Boehnke
Constructor University, Bremen, Germany
Editor: Daryl Swanepoel
Table of Contents
Table of Content
List of Tables
List of Figures
Executive Summary
1. Introduction
2. Measuring social cohesion
2.1 Data
2.2 Analytical approach
3. Level and trend of social cohesion
3.1 Social cohesion in South Africa
3.2 Social cohesion in the nine provinces
4. Structural influences on social cohesion
4.1 Data and method
4.2 Results
5. Individual experiences of social cohesion
5.1 Data and method
5.2 Four classes of experience
5.3 Socio-demographics of the four classes
6. Social cohesion and subjective well-being
6.1 Provinces
6.2 Individuals
7. Discussion and conclusion
References
Appendices
Appendix A: Indicators of cohesion across time
Appendix B: Dimensions of cohesion in the provinces over time
Appendix C: Correlations of social cohesion on the province level
Appendix D: Latent class analyses
Cover photo: istock.com - Stock photo ID:1440750455
List of Tables
Table 2.1 Sample sizes of Khayabus – Waves 1
Table 2.2 Factor loadings of items for dimensions within Domain 1, “Social relations”
Table 2.3 Factor loadings of items for dimensions within Domain 2 “Connectedness”
Table 2.4 Factor loadings of items for dimensions within Domain 3 “Focus on the common good”
Table 3.1 Social cohesion and its dimensions in South Africa across time
Table 3.2 The overall index of social cohesion in South African provinces across time
Table 4.1 Structural characteristics and social cohesion in South African provinces
Table 5.1 Social cohesion and its dimensions in the four classes
Table 5.2 Socio-demographic and economic characteristics of the four classes of respondents
Table 6.1 Social cohesion and subjective well-being in the provinces
Table 6.2 Subjective well-being in the four classes of respondents
Table A.1 Indicators of Dimension 1.1 “Social networks” across time
Table A.2 Indicators of Dimension 1.2 “Trust in people” across time
Table A.3 Indicators of Dimension 1.3 “Acceptance of diversity” across time
Table A.4 Indicators of Dimension 2.1 “Identification” across time
Table A.5 Indicators of Dimension 2.2 “Trust in institutions” across time
Table A.6 Indicators of Dimension 2.3 “Perception of fairness” across time
Table A.7 Indicators of Dimension 3.2 “Solidarity and helpfulness” across time
Table A.8 Indicators of Dimensions 3.2 “Respect for social rules” across time
Table A.9 Indicators of Dimension 3.3 “Civic participation” across time
Table A.10 Dimension 1.1 “Social networks” in the provinces across time
Table A.11 Dimension 1.2 “Trust in people” in the provinces across time
Table A.12 Dimension 1.3 “Acceptance of diversity” in the provinces across time
Table A.13 Dimension 2.1 “Identification” in the provinces across time
Table A.14 Dimension 2.2 “Trust in institutions” in the provinces across time
Table A.15 Dimension 2.3 “Perception of fairness” in the provinces across time
Table A.16 Dimension 3.1 “Solidarity and helpfulness” in the provinces across time
Table A.17 Dimension 3.2 “Respect for social rules” in the provinces across time
Table A.18 Dimension 3.3 “Civic participation“ in the provinces across time
Table A.19 Structural characteristics and social cohesion in South African provinces
Table A.20 Social cohesion and subjective well-being in the provinces
Table A.21 Goodness-of-fit indices of LCA solutions
Table A.22 Relative class sizes for LCA solutions
List of Figures
Figure 1.1 Constitutive elements of social cohesion (Leininger et al., 2021)
Figure 1.2 Measurement concept of the Bertelsmann Social Cohesion Radar
Figure 2.1 Provinces of South Africa
Figure 3.1 Overall index of social cohesion in South African provinces (2023)
Figure 3.2 Overall index of social cohesion in South African provinces across time
Figure 5.1 Average scores of dimensions in the four classes
Executive Summary
This study provides a comprehensive assessment of social cohesion in South Africa. It is an updated edition of the study published in November 2024, which focused on the development of social cohesion from 2021 to 2023, extending the period of observation to 2024.
Following the measurement concept developed by the authors and their colleagues for the Social Cohesion Radar of Bertelsmann Stiftung, the study assesses social cohesion in three domains: Social relations, Connectedness, and Focus on the common good. Each of these domains encompasses three dimensions of cohesion, namely: the intactness of Social networks, general Trust in people, and Acceptance of diversity within the domain Social relations; Identification with one’s place of residence, Trust in institutions, and Perception of fairness within the domain Connectedness; and Solidarity and helpfulness, Respect for social rules, and Civic participation within the domain Focus on the common good.
The study uses data from Waves 1 of the Khayabus Survey, a population-representative cross-sectional survey conducted annually by Ipsos South Africa. The Bertelsmann concept of defining a country’s level of social cohesion allows scores between 0 (no cohesion) and 100 (maximal cohesion). Which score can be regarded as sufficiently high is a normative, not to say political decision. It has become customary to designate scores below 20 as very low, from 20 to below 40 as low, from 40 to below 60 as moderate, from 60 to below 80 as high, and from 80 to 100 as very high. A very high level of social cohesion has not been reported for any country or other geopolitical entity yet.
In 2024, the overall level of social cohesion in South Africa as a whole was found moderate at 53.3 points. It went through a steady, though slow, decline from 53.5 points in 2021 to 51.7 points in 2023. By 2023, the downward trend in South Africa’s overall level of social cohesion has halted and reversed in an upward direction. The previously accrued decline has been almost overcome by 2024.
In 2024, the highest scores for a single dimension were found for Identification (72.2), followed by Solidarity and helpfulness (61.3), and Social networks (59.9). These dimensions constitute the glue that holds the South African society together. The lowest scores were found for Respect for social rules (36.6) and Perception of fairness (42.7). Although all nine dimensions improved from 2023 to 2024, only three have achieved an overall increase from 2021 to 2024: Trust in people (+2.0), Solidarity and helpfulness (+2.2), and Civic participation (+3.6). All other dimensions have registered an overall decline in the range from -0.2 for Acceptance of diversity to -3.8 for Respect for social rules.
As for the South African provinces, all exhibit moderate levels of social cohesion in 2024. In a comparison, cohesion was found lowest in KwaZulu-Natal (49.6), second lowest in North West (52.0), and third lowest in Free State (52.1). Cohesion was found highest in Limpopo (56.5). Over the period from 2021 to 2024, cohesion has improved only in North West (+1.2) and Eastern Cape (+2.6). All other provinces have registered an overall decline in the range from -0.1 for Gauteng to -4.2 for Northern Cape.
Correlational analyses on the provinces’ overall level of social cohesion and their structural characteristics offer insights on how to strengthen social cohesion: by enabling inclusive (people-centered) economic progress, reducing unemployment, lowering income inequality, providing adequate jobs for the highly qualified, promoting a family-oriented life-style, and bringing the living conditions in rural and urban areas to an equally adequate level.
The study, further, presents results from Latent Class Analysis (LCA), a grouping procedure that isolates distinct subgroups of South Africans who experience different strengths and deficits in social cohesion in their immediate life context. The preferred LCA solution identifies four classes of South Africans: Class 1 (Critics), which is characterized by high Identification but critically low Acceptance of diversity, a critically low perception of Respect for social rules, and low to moderate scores on the other dimensions; Class 2 (Integrated Sceptics), which is characterized by well-knit Social networks, high levels of Trust in people, Acceptance of diversity, Solidarity, and Civic participation, but low scores on the remaining dimensions; Class 3 (Middle South Africa), which is characterized by a high level of Identification with the country but moderate scores on all other dimensions; and Class 4 (Cohesive Communities), which is an ideal-typical model of strong cohesion in South Africa manifesting itself in exceptionally high Identification and high scores on all other dimensions. A series of chi-square tests of independence suggest that these four classes differ along core socio-demographic and socio-economic characteristics. The insights can help identify social groups at risk of experiencing low social cohesion.
Ultimately, the report examines the relationship between social cohesion and subjective well-being among South Africans. The evidence supports a core finding that has emerged in essentially all previous studies we have performed on social cohesion elsewhere: The quality of society (social cohesion) translates directly into citizens’ quality of life (subjective well-being). The analyses performed on the level of the provinces show that higher levels of social cohesion lead to a more positive evaluation of life, improved life satisfaction, and stronger optimism for the future. The same picture emerges from the analysis focusing on the four classes of respondents: In Class 4, Cohesive communities, families are predominantly seen as better off than a year ago, life satisfaction has improved for the majority, children are predominantly believed to have a bright future ahead.
It is exactly the strong positive relationship between social cohesion and subjective well-being that underscores the necessity of political action to improve South Africa’s level of social cohesion. Whereas conceptual academic work on social cohesion sometimes claims that too high a level of social cohesion can cement the societal status quo and prevent progress, all empirical studies have shown that high levels of cohesion foster peaceful coexistence of various societal groups in respect, dignity, trust, and cooperation. Cohesion translates the social and economic structures (performance and output of the economy, living conditions) into quality of life (happiness, life satisfaction) directly experienced by individual members of society. In case cohesion is neglected, one can expect societal polarization and political instability.
1. Introduction
Since the French Revolution with its famous motto “liberté, égalité, fraternité”, discourse on the cohesion of geopolitical entities (countries, provinces, neighborhoods) has seen waves of greater and lesser intensity, but one thing is clear: A healthy social entity needs fraternité or, in modern terminology, social cohesion among its members. Social cohesion stands for the ability of societies to stick together or, as Leininger and colleagues put it, “the glue that holds society together” (Leininger et al., 2021: 2).
In recent years, when social cohesion has been discussed in South Africa, it has been with an increasingly critical undertone. The sentiment that the self-declared Rainbow Nation (Tutu, Mandela) is drifting apart rather than growing together has become stronger. However, research – mainly empirical – on social cohesion in South Africa is scarce. Our search for any available scholarly literature within the past 10 years yielded seven publications, two of which are reviews of a book by Ballantine et al. (2017) included in the count. The book by Ballantine and colleagues is a collection of essays by local academics and public figures about issues related to, amongst others, inequality, xenophobia, safety, gender-based abuse, political leadership, law, education, identity, sport, arts, and South Africa’s position in the world. A paper by Abrahams (2016) tracks the evolution of social cohesion over twenty years in South African politics, criticizing the instrumentalization of cohesion as a social policy concept exclusively towards a form of nation-building that seeks to solidify the hegemony of the ruling party. A brief report by the South African Institute of International Affairs (2021) reviews the status quo and progress in religion, nationality, race and ethnicity, and LGBTQ+ rights. The latter report offers recommendations for improving the situation in these spheres and promotes the role of young people in fostering social cohesion. A paper by Burns and colleagues from the South African Labour and Development Research Unit emphasizes the importance of social cohesion as a social policy concept, also referring to studies conducted by the authors of the present report, critically reviews existing concepts of social cohesion proposed in the academic and policy discourse, and formulates a definition for its assessment in the South African society based on theoretical considerations: “Social Cohesion is the extent to which people are co-operative, within and across group boundaries, without coercion or purely self-interested motivation” (Burns et al., 2018, p. 10). Interestingly, Burns et al. (2018) identify the overlap between social cohesion and ubuntu, arguing that the two have become synonymous regarding nation-building and efforts to close South African society's cultural and racial divides.
To our knowledge, two existing empirical studies have defined and measured social cohesion in South Africa. Langer et al. (2017) define social cohesion in an African context as the interplay of three salient aspects: perceived inequalities, trust (interpersonal and institutional), and identity (national vs ethnic). Their measurement draws on data from 19 countries, including South Africa, from Round 3 (2005 – 2006), Round 4 (2008 – 2009), and Round 5 (2011 – 2013) of the Afrobarometer survey. For each aspect of a country, the authors calculate the proportion of respondents who provide those answers to the selected survey items that point to a stronger expression of cohesion. The resulting proportions for each aspect are then averaged by taking their arithmetic mean into a social cohesion index. According to the findings, overall cohesion in South Africa and its three aspects have recorded only minor ups or downs in the period studied. The level of identification was found to range from 0.612 (2005 – 2006) to 0.700 (2011 – 2013) and can be considered moderately high. The perception of equality was found in the range from 0.328 (2008 – 2009) to 0.469 (2011 – 2013) and can be qualified as low to moderately low. Trust was found in the range from 0.239 (2011 – 2013) to 0.293 (2005 – 2006) and can be qualified as low. From a comparative perspective, South Africa emerged in the middle of the country ranking on the overall level of cohesion and the perceived level of equality, in the lower half of the ranking on trust, and among the top countries on identification.
The second available empirical study (Leininger et al., 2021) similarly compares African countries. According to its authors, “cohesion is characterized by a set of attitudes and behavioural manifestations that includes trust, an inclusive identity and cooperation for the common good” (Leininger et al., 2021, p. 3). These three attributes unfold into two elements, encompassing horizontal or vertical relations among citizens and the state (see Figure 1.1): social trust and institutional trust, group identity and national identity, intergroup cooperation, and state-society cooperation. Leininger and colleagues operationalize their concept with items from the Afrobarometer, covering a varying set of African societies depending on the data availability for the particular element of cohesion: 17 to 18 in Round 3 (2005 – 2006), 20 in Round 4 (2008 – 2009), 28 to 34 in Round 5 (2011 – 2013), 32 to 36 in Round 6 (2014 – 2015). Their methodological approach measures the three attributes on a scale from 0 (low) to 1 (high). South Africa achieved scores in the range from 0.44 (2015) to 0.51 (2011) on trust, 0.3 (2015) to 0.43 (2006) on cooperation, and 0.42 (2015) to 0.74 (2011) on identity. These scores point to a downward trend in cohesion in South Africa for each attribute. In comparing African countries, South Africa ranked in the middle on trust, in the lower half on cooperation, and among the top countries on identity (except for the last year of observation, 2015). The work by Leininger et al. (2021), however, does not produce an overall index of cohesion, does not offer insights for more recent years since 2015, and does not venture into exploring the determinants of the country scores on the cohesion attributes or outcomes of cohesion.
Figure 1.1 Constitutive elements of social cohesion (Leininger)

Source: Leininger et al. (2021)
The present report attempts to close the gap in the existing research on South Africa. It aims to provide an all-around theoretically founded and methodologically sound empirical assessment of social cohesion in South African society. In particular, our study attempts to:
measure the current degree of social cohesion in South Africa and its nine constituent provinces;
track how cohesion has developed in the period from 2021 to 2024;
identify structural characteristics from the thematic fields of economic situation, inequality and poverty, demography, diversity, and modernization that promote or hinder social cohesion;
explore which social groups experience a high or low level of cohesion;
investigate how social cohesion, i.e., the quality of society, relates to citizens’ well-being, i.e., quality of life.
To achieve these aims, we apply the measurement concept of the Bertelsmann Social Cohesion Radar, which was informed by a comprehensive literature review (Schiefer & van der Noll, 2017) and the input of experts on the topic. It defines cohesion as the “quality of social cooperation and togetherness of a collective, defined in geopolitical terms, that is expressed in the attitudes and behaviors of its members. A cohesive society is characterized by resilient social relations, a positive emotional connectedness between its members and the community, and a pronounced focus on the common good” (Dragolov et al., 2016: 6). These three domains unfold into three dimensions. The domain Social relations measures the strength and resilience of individuals’ social ties (Dimension 1.1 – Social networks), the degree to which people trust others (Dimension 1.2 – Trust in people), and the extent to which people accept individuals of different background, lifestyle, and values as equal members of society (Dimension 1.3 – Acceptance of diversity). The domain Connectedness measures the strength of individuals’ identification with the geopolitical entity (Dimension 2.1 – Identification), the degree to which individuals trust the entity’s institutions (Dimension 2.2 – Trust in institutions), and individuals’ perception that they are treated fairly and that material resources are fairly distributed (Dimension 2.3 – Perception of fairness). The domain Focus on the common good captures the extent to which people feel and demonstrate responsibility for weak others (Dimension 3.1 – Solidarity and helpfulness), people’s willingness to abide by the rules of society (Dimension 3.2 – Respect for social rules), and their participation in society and political life (Dimension 3.3 – Civic participation). Figure 1.2 depicts the measurement concept of the Social Cohesion Radar (SCR).
Figure 1.2 Measurement concept of the Bertelsmann Social Cohesion Radar
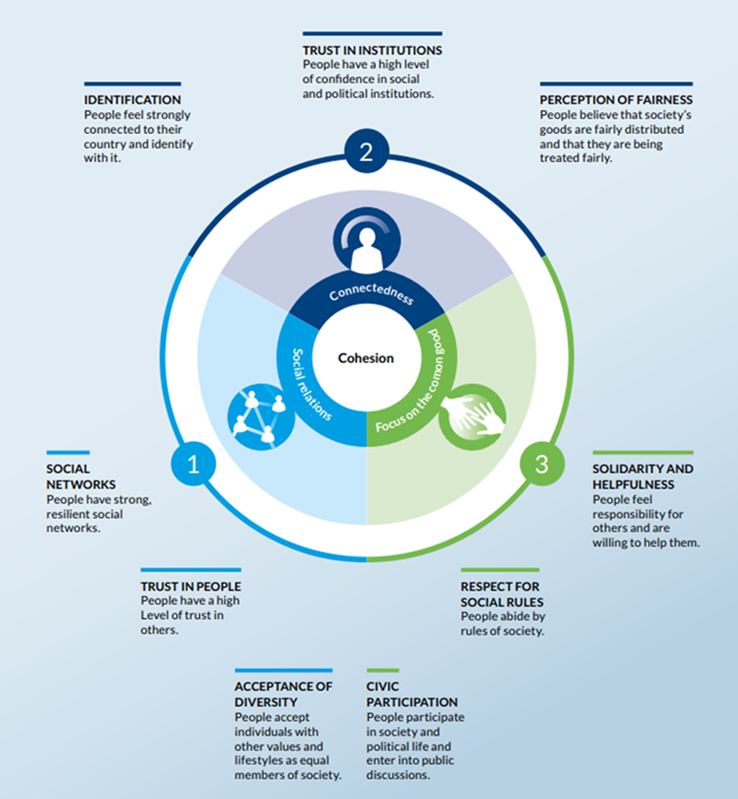
Source: Dragolov et al. (2016)
A comparison of the SCR to the approaches of Langer et al. (2017) and Leininger et al. (2021) shows that the three concepts overlap in several regards. First, social cohesion is a quantifiable quality of collectives, not individuals. Second, they all cover horizontal and vertical ties. Third, they all suggest that social cohesion should be assessed via a perception-based index, not based on objective socio-demographic indicators. Next, there is a considerable conceptual overlap in the emphasis on specific dimensions (aspects) of social cohesion. As already mentioned above, Langer et al. suggest including the extent of perceived inequalities (SCR: Perception of fairness), the societal level of trust (SCR: Trust in people), and the strength of people’s adherence to their national identity (SCR: Identification). Leininger and colleagues also mention trust and identity, whereas their dimension of cooperation is called Solidarity and helpfulness in the SCR.
The main difference between the three approaches lies in their conceptual scope. Whereas the SCR comprehensively describes the components necessary for a full-fledged assessment of the level of social cohesion in a given society, the two concepts based on the Afrobarometer remain somewhat piecemeal. In addition, one of the main advantages of the SCR approach is its leanness, a necessity also underscored by Leininger et al. (2021). On the one hand, the concept covers the essential components of social cohesion. At the same time, it leaves room for a systematic exploration of determinants (e.g., state of the economy, socio-economic exclusion) and outcomes (e.g., population well-being). For a critical review of the advantages and disadvantages of measurement concepts of cohesion that have been applied in empirical research, we refer readers to Delhey, Dragolov, and Boehnke (2023).
On a final note, the SCR has been utilized to assess social cohesion in 34 Western (EU and OECD) countries (Dragolov et al., 2016), 22 Asian countries (Delhey & Boehnke, 2018), the 16 federal states of Germany (Dragolov et al., 2016), 79 spatial planning regions of Germany (Arant et al., 2017; Boehnke et al., 2024), 78 neighborhoods of the Free Hanseatic City of Bremen (Arant et al., 2016), the 32 federal entities of Mexico (Boehnke et al., 2019), and the seven regions of Kyrgyzstan (Larsen & Boehnke, 2016).
2. Measuring social cohesion
This section details the data and methodological approach employed for measuring social cohesion in South Africa.
2.1 Data
The current report offers empirical evidence from analyses performed on data from the Khayabus Survey. The data were collected by Ipsos South Africa and provided by the Inclusive Society Institute. The Khayabus Survey is a population-representative cross-sectional survey that has been fielded annually in at least two waves among respondents of age 15 and above. The survey covers various topics related to society and politics in South Africa. It initially included the sections Socio-Political Trends (SPT), Government Performance Barometer (GPB), and Party Image (PI). A fourth section, GovDemPol, was added in 2021.
Because the GovDemPol section includes the core set of indicators needed for assessing social cohesion along the concept of the Social Cohesion Radar, our analyses can only start with 2021. Data on three items crucial for the measurement concept, each belonging to the SPT section, had to be taken from the 2020 Khayabus, as they were not included in the 2021 survey. The most recent survey data available to us refer to the year 2024. Our report concentrates on survey respondents aged 18 and above, as several of the needed items were not included in the questionnaire for under-aged South Africans. The analyses performed for this report draw on Waves 1 of the Khayabus survey, typically conducted from late May/early June to mid-July. The overall samples in the employed data encompass 3758 respondents in 2020, 3402 respondents in 2021, 3459 respondents in 2022, 3519 respondents in 2023, and 3172 respondents in 2024.
Table 1.1 Sample sizes of Khayabus – Waves 1
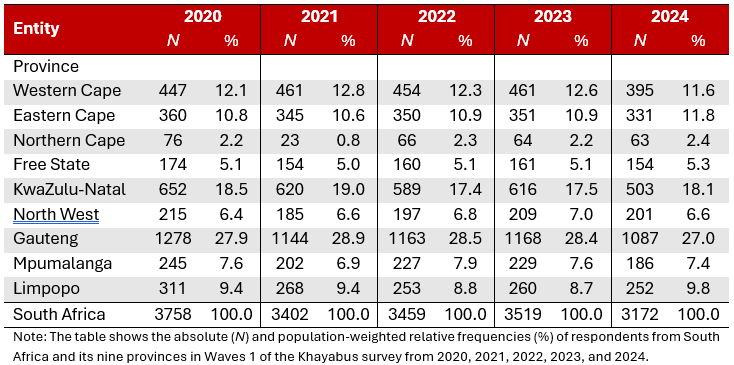
Table 2.1 offers detailed information on sample sizes achieved in Waves 1 of the Khayabus survey from 2020 to 2024. The table provides a breakdown of the samples by province, as the present report also aims to measure social cohesion in the nine constituent provinces of South Africa. We refer readers unfamiliar with the nine provinces' geographic location and administrative borders to Figure 2.1. It is important to note that population sizes vary widely between the mostly urban Gauteng province, where well over a quarter of South Africa's adult population lives, and the mostly desert Northern Cape province, which encompasses less than 3%. The uneven distribution of the South African population across the provinces is reflected in the achieved sample sizes for the provinces, as evident from Table 2.1. The low sample sizes for the least populated provinces, e.g., Northern Cape with typically about 60 respondents per wave, do not necessarily reduce the representativity of the data concerning core socio-demographic characteristics of their population. We address this issue by calibrating the survey data with the population weights provided by Ipsos South Africa. Smaller sample sizes do, however, involve a larger standard error for sample statistics like percentages and means. In practical terms, this means that the precision of the measurements for Gauteng (NGP,2024 = 1087) is about four times higher than that for Northern Cape (NNC,2024 = 63) at the same variability in the data. Caution is, therefore, required when interpreting such statistics as estimates of the ‘true’ situation or opinion in the population of provinces for which low sample sizes are available.
Figure 1.3 Provinces of South Africa

Source: Apraku et al. (2018)
In addition to the above-addressed statistical issues, population sizes, and population density are closely related: In the Gauteng province, more than 800 people live per square kilometer, whereas in the Northern Cape province, the density figure is below 4 per square kilometer (Statistics South Africa, 2024a). Considering these stark differences is essential when evaluating our findings on levels and trends of social cohesion in South Africa.
2.2 Analytical approach
Below we elaborate on the methodological approach for assessing social cohesion. We begin with the strategy for selecting Khayabus survey questions, also referred to here as items or indicators, to measure the nine dimensions of social cohesion in line with the Bertelsmann concept. We then turn to the approach for computing scores for the nine dimensions and the overall social cohesion index.
Item selection was conducted using a multi-step procedure. First, members of the research team – independent of each other – identified potential items for measuring the nine cohesion dimensions from the Khayabus questionnaire according to face validity. Members of the research team then jointly prepared a pool of items according to face validity. In the third step, items from the pool were subjected to an exploratory factor analysis for each dimension. Factor analysis is a statistical sorting procedure that analyzes the matrix of item intercorrelations to separate items with a highly similar response pattern from items with a different response pattern and then sort them into distinct subgroups. The various subgroups of items (called factors) allow us to assess whether or not the items were selected appropriately according to their face validity as per the different dimensions of social cohesion. An important selection criterion is the item’s factor loading, which reflects how strongly an item is correlated with the other items sorted into the given factor. Item loadings should typically exceed .40 to be seen as sufficiently high. Items exhibiting sufficiently high factor loadings were retained. In the final step, we assessed the internal consistency of the scales formed by the selected items to measure a pertinent dimension. Cronbach’s α consistency coefficients should reach .90 for an excellent scale, .80 for a very good scale, .70 for a satisfactory scale, and minimally .30, or, in case of short scales, at least .10 times the number of items in the scale.
Several data preparation steps had to be taken before performing factor analyses. Where needed, the response options of the items were reverse coded so that a higher numerical value stands for a more vital expression of the pertinent aspect of cohesion. The response options of all items were rescaled to range from 0 (weakest expression of cohesion) to 100 (strongest expression of cohesion). If present, missing values on an item were substituted with the sample mean as the missingness rate was very low. Tables 2.2 to 2.4 document the selected items' factor loadings and the internal consistencies of the scales these items form for measuring the nine dimensions of social cohesion.
Table 1.2 Factor loadings of items for dimensions within Domain 1 “Social relations”
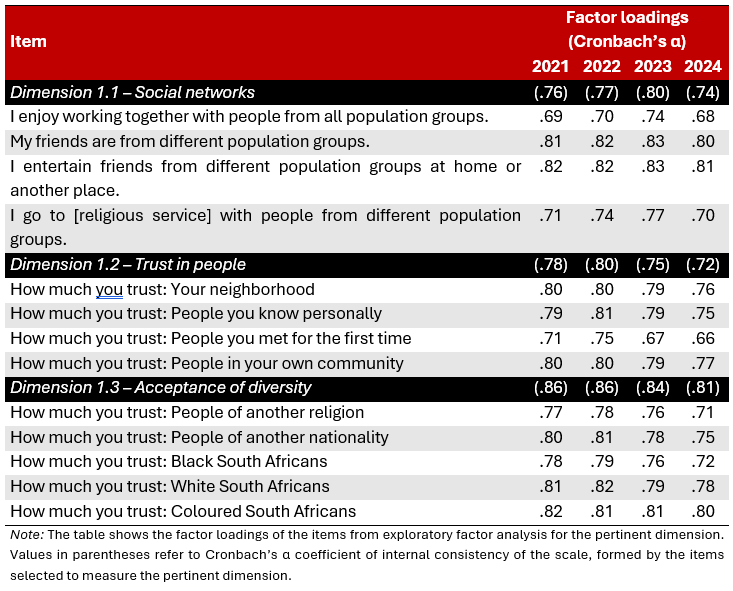
Table 1.3 Factor loadings of items for dimensions within Domain 2 “Connectedness”
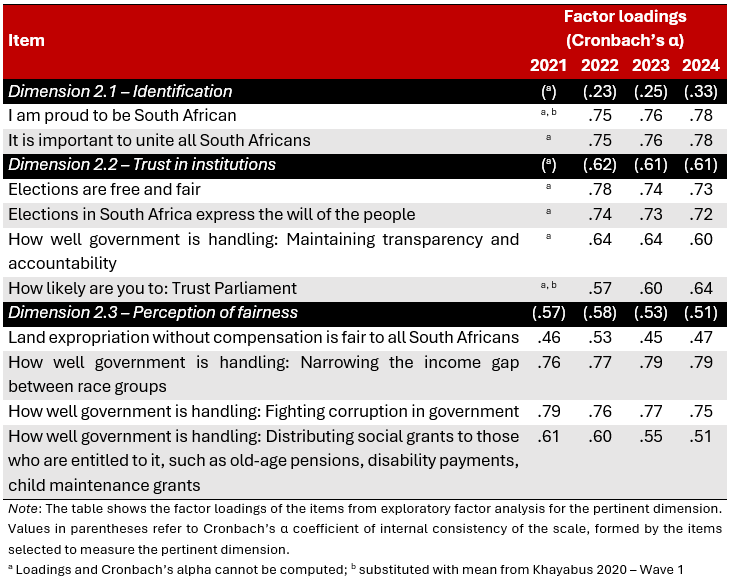
Table 1.4 Factor loadings of items for dimensions within Domain 3 “Focus on the common good”
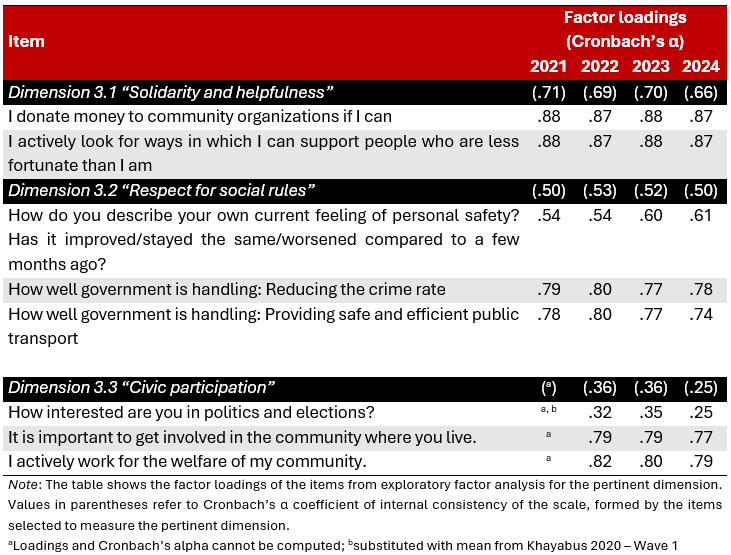
Readers will note that not all dimensions of social cohesion were measured equally well. This had several reasons. To begin with, the questionnaire offered a limited choice of indicators for some dimensions. This is why not all dimensions could be measured with at least three items. This pertained to Dimension 2.1 (Identification) and Dimension 3.1 (Solidarity and helpfulness), for which only two items could be included. Moreover, for Dimension 2.1, an item from the 2020 Khayabus had to be included, even with two items, to assess citizens’ identification with South Africa in 2021. Second, not all scales exhibit a high degree of homogeneity (level of intercorrelation) of the included items. This is particularly true for Dimension 2.1 and Dimension 3.3 (Civic participation).
After sorting items via factor analyses, the nine dimension scores were computed by calculating the arithmetic mean of the items determined to belong to a given factor. The overall cohesion index was calculated as the arithmetic mean of the nine dimension scores. Dimension and index scores for the provinces and South Africa were calculated by aggregating the individual-level data to the respective level via the population-weighted arithmetic mean. Scores for the dimensions and the overall index range from 0 (very low cohesion) to 100 (very high cohesion), where scores from 0 to 19.99 can be interpreted as pointing to a very low level of cohesion, 20 to 39.99 – low, 40 to 59.99 – medium, 60 to 79.99 – high, and 80 to 100 – very high.
3. Level and trend of social cohesion
In this section, we report findings on the level and trend of social cohesion in South Africa and its nine constituent provinces from 2021 to 2024.
3.1 Social cohesion in South Africa
Table 3.1 documents the annual level and trend of social cohesion in South Africa since 2021. We first present the findings for 2024, the most recent year for which data are available, and then proceed to the changes observed over time.
Level in 2024
In 2024, the overall social cohesion index for South Africa was 53.3, slightly above the theoretical midpoint of the measurement scale of 50. As such, the strength of social cohesion in South Africa can be qualified as moderate—neither high nor low. What is behind this result? A look at the single dimensions reveals the strong and weak spots of cohesion in South Africa that jointly produce its moderate overall level.
Table 1.5 Social cohesion and its dimensions in South Africa across time
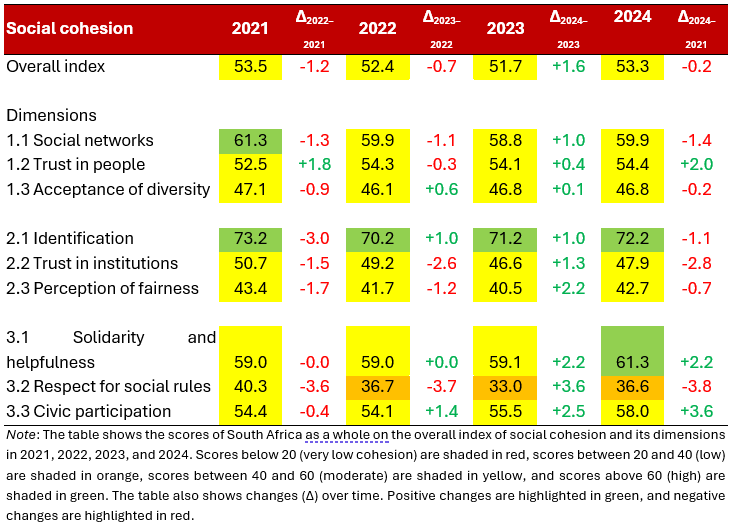
Dimension 1.1, Social networks, scored 59.9 in 2024. The intactness of citizens’ social networks is currently the third strongest dimension in South Africa. The strength of this dimension can be qualified as moderate to high as it has almost reached the lower bound of the interval of high scores (60). To exemplify with one indicator for this dimension: About 52.5 % of the respondents agreed with the statement “I entertain friends from different population groups at home or another place,” 24.8 % disagreed, and 20.7 % positioned themselves in between (see Table A.1 of the Appendix).
Dimension 1.2, Trust in people, can also be found in 2024 in the upper half of the measurement scale, with a score of 54.4. The level of trust that South Africans place in others qualifies, thereby, as moderate. To exemplify the finding with one indicator for this dimension: 17.7 % of the respondents trust people in their community completely, 64.6 % only somewhat or not very much, and 16.5 % not at all (see Table A.2).
Dimension 1.3, Acceptance of diversity, achieved in 2024 a score of 46.8. The numeric result qualifies the tolerance level in South African society still as moderate. However, it should be noted that it falls within the lower half of the measurement scale, unlike the previous two dimensions from the domain Social relations. To exemplify with one indicator for Acceptance of diversity: 10.8 % of the respondents trust coloured South Africans completely, 58.9 % only somewhat or not very much, and 28.5 % not at all (see Table A.3).
Dimension 2.1, Identification, scored 72.2, the highest among all dimensions in 2024. Identification is the most pronounced aspect of social cohesion in South Africa and the only dimension that can be qualified without any reservations as high. This is manifested, for example, in the responses to the statement “I am proud to be South African”: 75.4 % agreed, 13.9 % disagreed, and 9.2 % positioned themselves in between (see Table A.4).
Dimension 2.2, Trust in institutions, achieved in 2024 a score of 47.9. Just like Acceptance of diversity, the extent of trust citizens have in the country's institutions can be considered moderate. However, it falls within the lower half of the measurement scale. To exemplify with one indicator: 48.7 % of the respondents agreed with the statement “Elections are free and fair,” 30.6 % disagreed, and 17.3 % positioned themselves in between (see Table A.5).
Dimension 2.3, Perception of fairness, scored in 2024 at 42.7. It is the second weakest aspect of social cohesion in South Africa. The extent to which people perceive the distribution of material resources as fair can be qualified as moderate. However, it should be noted that the result is very close to the upper bound of the interval of low scores (40). To exemplify with one indicator for this dimension: 29.9 % of the respondents stated the government is doing very well or fairly well at narrowing the income gap between races, whereas 65.3 % stated the government is handling this issue not very well or not at all well (see Table A.6).
Dimension 3.1, Solidarity and helpfulness, achieved in 2024 a score of 61.3. With this result, it is the second most vital aspect of social cohesion in South Africa. The extent to which people help the weak members of society can be qualified as high. To exemplify with one indicator for this dimension: 60 % of the respondents agreed with the statement “I actively look for ways in which I can support people who are less fortunate than I am”, 18 % disagreed, and 20.4 % positioned themselves in between (see Table A.7).
Dimension 3.2, Respect for social rules, achieved in 2024 a score of 36.6. This is the weakest aspect of social cohesion in South Africa. The extent to which people perceive that rules are observed is low. To exemplify with one indicator for this dimension: 23.5 % of the respondents stated that the government is doing very well or fairly well at reducing the crime rate, whereas 74.9 % stated not very well or not at all well (see Table A.8).
Dimension 3.3, Civic participation, scored at 58.0 in 2024. Citizens’ involvement in society and political life can be qualified as moderate, tending towards high. One indicator for this dimension: 51.4 % of the respondents agreed with “I actively work for the welfare of my community”, 28.5 % disagreed, and 18.2 % were undecided (see Table A.9).
The results for the dimensions form a nuanced profile of cohesion. None of the three domains of social cohesion (Social relations, Connectedness, and Focus on the common good) exhibits either only deficits or only strengths for all its dimensions. Interestingly, the three top-scoring dimensions (Identification, Solidarity and helpfulness, and Social networks) stand out as strong to moderately strong anchors of their respective domains. However, two domains are imbalanced: Connectedness by the moderately low Perception of fairness, and Focus on the common good by the low Respect for social rules. If one should point out the glue that holds South African society together, this would undoubtedly be citizens’ strong identification with the country, their solidarity with their weaker fellow citizens, and the functioning of their social networks. On the other hand, what could destabilize the South African society is the perceived lack of respect for rules and the perceived lack of distributional fairness.
Trend over time
Regarding the trajectory of social cohesion, the results summarized in Table 3.1 present a mix of good and bad developments. To begin with the good, the year 2023 marks an inflection point in the downward trend of the overall index that had been observed since 2021. Following a steady, though slow, decline from its level at 53.5 in 2021 down to 52.4 in 2022 and further down to 51.7 in 2023, the overall index of social cohesion has increased to the current level of 53.5. The overall index has, thereby, recovered by 1.6 points from 2023 to 2024, but not fully yet to the level measured in 2021, as it is still -0.2 points below this benchmark.
The almost full recovery of the overall index of social cohesion should not be overestimated as it masks diverging trajectories among the dimensions. It is indeed the case that all nine dimensions increased from 2023 and 2024. The gains vary considerably from +0.1 points for Acceptance of diversity to +3.6 points for Respect for social rules. For six dimensions, the increase over the past year does not suffice to compensate for the previously accrued decline. The latter issue is most pronounced for Respect for social rules. In 2021, this dimension ranked as moderate with a borderline score of 40.3 but lost -7.3 points over 2022 and 2023 to qualify in the low-level category, where it can still be found despite the halving of the decline from 2023 to 2024. In fact, Respect for social rules in South Africa is approximately back to its level from 2022. A similar trajectory can be observed for Social networks: From an initially high level of 61.3, over 2022 and 2023 it slid down by 2.4 points to the moderate-level category, and is still to be found there in 2024 despite a gain of +1 point up to its current level, which happens to be identical to that from 2022. Other dimensions that are currently still below their starting positions from 2021 are Trust in institutions (-2.8 points), Identification (-1.1 points), Perception of fairness (-0.7 points), and Acceptance of diversity (-0.2 points).
The remaining three dimensions follow the opposite trajectory. By 2024, they have not just made up for declines experienced prior to 2023, but have, moreover, surpassed their starting levels from 2021. The most pronounced increase can be observed for Civic participation (+3.6 points), followed by Solidarity and helpfulness (+2.2 points), and Trust in people (+2.0 points). Further, Solidarity and helpfulness has already made it into the high-level category. We refer readers to Tables A.1 to A.9 of the Appendix for changes over time in the responses to the indicators of the respective dimensions.
3.2 Social cohesion in the nine provinces
Level in 2024
Zooming into the provinces, we find some variation across these administrative units. Figure 3.1 maps the strength of the overall social cohesion index in the nine provinces. Table 3.2 documents the annual level and trend over the four years examined here. In 2024, social cohesion was found relatively lowest in KwaZulu-Natal (49.6), second lowest in North West (52.0), and third lowest in Free State (52.1). Mpumalanga (53.2) comes closest to the country average of 53.3. Cohesion is higher than the result for South Africa as a whole in Gauteng (54.0), Eastern Cape (54.4), Western Cape (54.5), Northern Cape (55.1), and highest in Limpopo (56.5). In fact, all provinces rank in the interval of the measurement scale, referring to a moderate level of cohesion.
Figure 1.4 Overall index of social cohesion in South African provinces (2024)

Note: The map applies the colour scheme displayed above to visualize the strength of social cohesion in 2024 across the nine provinces.
Table 1.6 The overall index of social cohesion in South African provinces across time
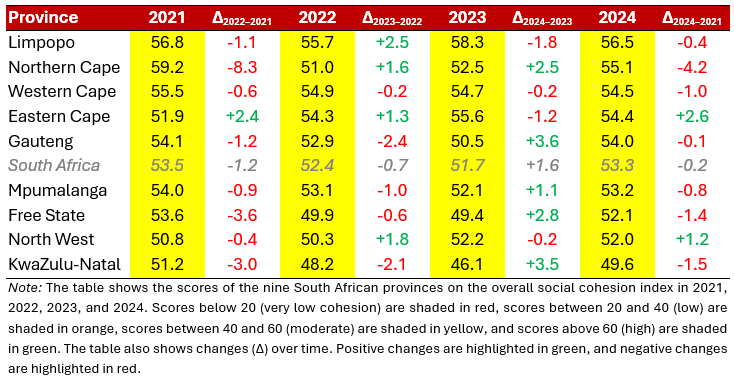
Tables A.10 to A.18 of Appendix A document the provinces' performance on the single dimensions of cohesion. Interestingly, none of the provinces exhibits a consistent profile. Each province has a distinctive pattern of strengths and weakness which is evident both in an absolute and in a relative comparison of the provinces’ dimension scores. The only exception would be the strength of identification with the country, which is, in absolute terms, high across all provinces. To exemplify the idiosyncrasies of the provinces, we take Limpopo and KwaZulu-Natal, the two extremes in the ranking on the overall index of cohesion. Limpopo, achieves the relatively highest scores on altogether five dimensions from the domains Connectedness and Focus on the common good, but shows deficits on two dimensions from the domain Social relations. In particular, Limpopo occupies the second lowest rank on Trust in people, found highest in Eastern Cape (61.0), with a moderate score of 48.1, and the lowest rank on Acceptance of diversity, found highest in Gauteng (49.6), with a low score of 37.9. On the other hand, KwaZulu-Natal occupies bottom ranks on six dimensions across all three domains but achieves the second highest score on Trust in people (57.2).
Trend over time
As evident from Table 3.2, social cohesion in the provinces has followed five different trajectories from 2021 to 2024. The most common trajectory comprises a larger decline in the beginning followed by a smaller increase, which, taken together, result in an overall decline. Readers may recall that this is the trajectory of South Africa as a whole on the overall index of cohesion and all dimensions but Trust in people. It applies to five provinces: Northern Cape (-4.2 points), KwaZulu-Natal (-1.5 points), Free State (-1.4 points), Mpumalanga (-0.8 points), and Gauteng (-0.1 points). The opposite trajectory – a larger increase in the beginning and a later smaller decline – applies only to Eastern Cape (+2.6 points). Two other provinces, Limpopo and North West, have experienced a zig-zag trajectory similar to that of South Africa as a whole on Trust in people: a decline followed by an increase and then another decline. Due to differences in the magnitudes of these ups and downs, the combined effect is an overall decline in Limpopo (-0.4 points) and an overall increase in North West (+1.2 points). Finally, a steady decline characterizes the trajectory of cohesion in Western Cape, resulting in an overall decline of -1 point. Taken together, the five trajectories bring about an overall decline of social cohesion in seven provinces and an overall increase in only two. Figure 3.2 depicts these developments in the overall cohesion index across the provinces.
Readers may notice in Table 3.2 that the divergence of the provinces observed from 2022 to 2023 has reversed to a convergence from 2023 to 2024. The range of the provinces’ scores on the overall index, i.e. the difference between the top ranked and the bottom ranked province, has decreased from 12.2 points in 2023, when it was the largest across all years analyzed, to only 6.9 points in 2024. In fact, the range in 2024 is the smallest observed in the period from 2021 to 2024. The closing gap in the strength of cohesion among the provinces is, however, misleading. Behind it is not a process of upward convergence, i.e. former ‘losers’ catching up, but one of downward convergence, namely the weakening cohesion in Limpopo and Northern Cape.
Figure 1.5 Overall index of social cohesion in South African provinces across time

Note: The figure shows the scores of the nine provinces on the overall index of social cohesion in 2021, 2022, and 2023.
As to the trend in the single dimensions, Tables A.10 to A.18 reveal that Social networks, Solidarity and helpfulness, and Civic participation are the only dimensions that have improved for almost all provinces. Social networks have weakened only in KwaZulu-Natal (-1.3 points), and more pronouncedly in Gauteng (-5.1 points) and Mpumalanga (-8.8 points). Solidarity and helpfulness has declined only in Western Cape (-3.1 points), Northern Cape (3.2 points) and more dramatically in Mpumalanga (-12.7 points). The only declines observed on Civic participation affect North West (-0.5 points) and Northern Cape (-1.1 points). It is worrying that the picture is reversed for Identification, which holds the South African society together: Whereas citizens’ strength of identification has improved in Limpopo (+6.5 points) and North West (+6.6 points), it has declined in the remaining seven provinces in the range from -0.2 points (Eastern Cape) to -5.9 points (Free State). Perception of fairness and Respect for social rules – the major weaknesses of social cohesion in South Africa – as well as Trust in institutions also register predominantly declines across the provinces. Respect for social rules has returned to its initial level from 2021 in Limpopo, and has increased only in Northern Cape (+1.4 points) and Western Cape (+1.9 points). We observed the largest decline in Respect for social rules in KwaZulu-Natal (-11.6 points), despite an improvement of +2.6 points from 2023 to 2024. Perception of fairness has improved only in Western Cape (+0.2 points), Gauteng (+1.8 points), and Limpopo (+6.2 points). The extent to which citizens place trust in institutions has improved only in Mpumalanga (+1.2 points), Western Cape (+2.1 points), and Northern Cape (+2.3 points). Following a steady downward trend, Trust in institutions has declined by -11.7 points in KwaZulu-Natal. On a final note, Trust in people and Acceptance of diversity are also characterized by more provinces being on a decline, although the ratio here is rather balanced at 5:4. It is noteworthy, though, that Limpopo has experienced a steady decline across all years both on the extent to which citizens trust others (-9.8 points) and the extent to which citizens accept diversity (-12.9 points). Acceptance of diversity has consistently declined also in Western Cape (-6.5 points). In contrast, we observe a steady increase on Trust in people for Eastern Cape (+11.6 points) and North West (+9.5 points), and a steady increase on Acceptance of diversity in Mpumalanga (+12.3 points).
In the subsequent section, we investigate which structural characteristics of the provinces may be at play in promoting or hindering social cohesion.
4. Structural influences on social cohesion
This section aims to find evidence on structural determinants of social cohesion. For this purpose, we explore the relationship between the overall level of social cohesion in the nine provinces and selected characteristics of the provinces from the following thematic fields: economic situation, inequality and poverty, demography, diversity, and modernization. The focus on these aspects is not arbitrary: Our studies on Western and Asian societies as well as the federal states and spatial planning regions of Germany demonstrated empirically that aspects from these thematic fields act as determinants rather than outcomes of social cohesion (Dragolov et al., 2016; Bertelsmann Stiftung, 2018; Arant, Dragolov & Boehnke, 2017; Boehnke, Dragolov, Arant & Unzicker, 2024).
4.1 Data and method
To touch on the economic situation in the provinces, we use data on the gross domestic product per capita[1] in Rand (Statistics South Africa, 2024a), Human Development Index (Global Data Lab, 2024), and unemployment rates – official and expanded (Statistics South Africa, 2023b). We measure poverty using one subjective indicator – the share of households in a province who perceive themselves as poor (Statistics South Africa, 2024c), and objective indicators concerning three definitions of the poverty line[2] – the share of the population below the food poverty line, the lower-bound poverty line, and the upper-bound poverty line (own calculations based on CRA, 2023). We employ the Gini index of income inequality and the P90/P10 ratio (own calculations based on CRA, 2023) to measure inequality[3]. We draw on data from Census 2022 (Statistics South Africa, 2023a) for the remaining thematic fields. In particular, as to demographics, we consider population density, the share of urban and rural population, the share of singles and married citizens, and the population's median age. To tap into diversity, we use the share of Blacks, Whites, Coloured, Indian/Asian, and Other races; the share of immigrants; as well as ethnic, linguistic, and religious fractionalization[4] (own calculations based on Statistics South Africa, 2023a). To touch on modernization, we use the share of citizens with completed primary, secondary, and post-secondary education, the share of citizens owning a computer and a cell phone, and the share of the population without access to the internet.
For reasons of data availability, all structural indicators refer to the years 2021 or 2022. Thereby, they precede the most recent measurement of social cohesion from 2024 by about two to three years. The intentional time lag—earlier measurement of the provinces’ structural characteristics and later measurement of social cohesion—introduces a certain degree of temporal order in the analyses. It cannot prove the existence of a causal relationship, but it can increase the plausibility of attributing causality.
Each of the above-listed structural characteristics of the provinces was subjected to a correlation test with the level of social cohesion. Two variables are correlated when changes in one are (closely) followed by changes in the other. A correlation can be positive (the more of Variable A, the more of Variable B) or negative (the more of Variable A, the less of Variable B). The strength of the association is reflected in the correlation coefficient, which can range from 0 (no correlation) to ±1 (perfect correlation). Typically, a correlation of size below |.10| is very weak and not worth interpreting, between |.10| and |.30| – weak, between |.30| and |.50| – moderate, and above |.50| – strong.
A considerable obstacle arises from the sample size for the correlations on the level of provinces. Of course, the overall sample size in each survey wave is large and has sufficient statistical power. Power (in mathematical-statistical theory) means that a given sample is large enough to corroborate a particular effect as likely ‘true’ about the population from which the sample was drawn – South Africa in this case. On the level of provinces, however, showing that a specific correlation is sizable enough to conclude that it is significant (i.e., likely true in the nine provinces) is problematic due to the number of provinces – only nine, unlike the number of surveyed individuals – over three thousand in each year. This may be surprising at first glance because more people have been surveyed in the provinces, with a minimum of 63 in Northern Cape and a maximum of 1087 in Gauteng in 2024. However, social cohesion is not a characteristic of individuals but of geopolitical entities, and the latter – the provinces of South Africa – are only nine.
The low number of cases (provinces) means that only extremely high correlations can reach statistical significance. Mathematically, significance is a function of sample size (the higher, the more likely it is for a particular coefficient to be significant) and data variability (the higher the variance of the included data, the less likely it is that a specific coefficient is significant). Given these constraints, we disregard the significance of the correlation coefficients in our reporting and interpretation, focusing instead on the tendency in the data. Thus, we follow the appeal of a respectable number of scientific community members to ditch p-values (Wasserstein, Schirm & Lazar, 2019). The results from additionally performed bivariate biserial Pearson correlations, for which the province-level characteristics were disaggregated to the individual-level data set for 2024 (N = 3172), show that ten of altogether 31 associations may not be considered significant (see Table A.19 of Appendix C). This finding supports our decision to focus on tendencies instead of discarding associations merely for not meeting a criterion for statistical significance.
Besides significance, the number of provinces is critically low for performing Pearson correlations. As a parametric test, the Pearson correlation involves assumptions that cannot be fulfilled with the data on the level of provinces. We, therefore, resort to Spearman correlations as a non-parametric, assumption-free alternative. A Spearman correlation is, in essence, a Pearson correlation performed on ranked data. The distinction between the two approaches is that a Pearson correlation considers the exact distances among the observations on each variable, whereas a Spearman correlation considers only whether there are differences, disregarding their size. For example, in 2022, the wealthiest province in terms of per capita GDP was Gauteng, with 96,252 Rand, and the poorest was Eastern Cape, with 54,805 Rand. The Pearson correlation will be influenced by the numeric difference of 41,447 Rand between the two provinces, whereas the Spearman correlation will only consider which province has the higher value. One implication is that the Spearman method is not sensitive to outliers in the data – observations (provinces) with an extremely large or extremely low value on a characteristic of interest. Given the critically low sample size of nine provinces, the Spearman method is advantageous compared to a Pearson correlation which may be quickly and heavily biased by an outlier. Because in all our previous studies on cohesion, the data allowed us to apply the Pearson correlation method, we performed Pearson correlations for the present report, too. Interested readers can find those in Table A.19 of Appendix C. In a few instances, we observe great discrepancies – mostly in size but also in direction. As discussed at length above, to stay on the safe side, we report and interpret the findings from the Spearman correlation tests.
On a final note, we performed both bivariate correlations and partial correlations for GDP, because both in our study of 34 Western countries (Dragolov et al., 2016) and our study of 22 Asian countries (Bertelsmann Stiftung, 2018), GDP was highly positively related to social cohesion: The more prosperous a society, the more cohesive it is. Partialling GDP out of a relationship removes the influence of GDP on both variables involved. This makes it possible to speak of associations between a given structural characteristic of the provinces and social cohesion, independent of their economic prosperity. In the section below, we report and interpret the partial correlations.
4.2 Results
Table 4.1 documents the relationships between the structural characteristics of the nine provinces and social cohesion per thematic field. The empirical findings for South Africa present several surprises concerning what has been previously found for Western and Asian societies.
Economic situation
The bivariate correlation between per capita GDP (in a province) and social cohesion (in the same province) was found at ρ =.00. The relationship is non-existent. It informs that social cohesion is independent of how economically affluent the provinces are. This result is striking as it goes against the positive association between GDP and cohesion that was consistently found in all our previous studies on Western and Asian societies.
The correlation between the Human Development Index and cohesion emerged positive and moderate in size (ρ = .39). People-centered economic progress (Gross National Income coupled with mean years of schooling and life expectancy) appears conducive to social cohesion.
Both measures of unemployment – the official and expanded unemployment rates – exhibited moderately negative associations with social cohesion (ρ = -.42 and ρ = -.32, respectively). Provinces in which more unemployed people reside tend to have weaker social cohesion.
Poverty and inequality
The evidence presents mixed findings on poverty. Whereas the subjective indicator exhibited a strongly negative correlation with social cohesion (ρ = -.55), the three objective indicators were found to correlate positively but only very weakly in the range from ρ = .03 for the upper-bound poverty line to ρ = .10 for the food and lower-bound poverty lines. Social cohesion tends to be lower in provinces where more households perceive themselves as poor. Its level, however, appears to be, by and large, unrelated to objective poverty. Though at first glance puzzling, the results on objective poverty can be explained with the efficiency of social welfare programs targeted at supporting poor citizens.
The correlations of the social cohesion index with both measures of income inequality were found to be consistently negative. Social cohesion tends to be lower in provinces with larger inequality in income. Interestingly, the correlation with the Gini index is moderately negative (ρ = -.44) and insignificant, whereas that with the P90/P10 ratio is strongly negative (ρ = -.65) and marginally significant (p ≤ .10). The Gini index considers the entire income distribution. In contrast, the P90/P10 ratio contrasts the income at the top of the distribution (90th percentile) to the income at the bottom (10th percentile). The latter focuses on inequality, which is more visible and more accessible for ordinary citizens to perceive. The top-to-bottom income ratio is 38 in the Free State and 11 in the Northern Cape. These values inform that the top earners' income is 38 times higher than that of poor citizens in the Free State; in Northern Cape – ‘only’ 11 times higher. Vast discrepancies in income emerge as detrimental to social cohesion.
Table 1.7 Structural characteristics and social cohesion in South African provinces

Demography
Population density exhibited a weak negative relationship with social cohesion (ρ = -.22). Social cohesion tends to be lower in more densely populated provinces. This finding corresponds with the associations of the cohesion index with the shares of urban (ρ = -.30) and rural population (ρ = .30). Though the relationships are weak in size, they indicate that social cohesion tends to be lower in more urbanized provinces and, in contrast, higher in provinces with a larger share of rural population.
Marital status was found to correlate strongly with social cohesion. Social cohesion appears to be significantly lower in provinces with a large share of singles (ρ = -.69, p ≤ .10) and tends to be higher in provinces with a larger share of married citizens (ρ = .47). This finding suggests that families contribute strongly to cohesion in the South African society.
We found a moderately negative association (ρ = -.44) with median age. Social cohesion tends to be lower where the population’s median age is higher. The association was reversed in Asia: Social cohesion was found higher in Asian countries with a higher median age.
Diversity
The composition of the provinces’ population regarding race, migration background, language, and religion seems to be weakly to moderately related to social cohesion. Provinces with larger shares of Blacks (ρ = -.16) tend to have a somewhat weaker level of social cohesion. In contrast, cohesion tends to be somewhat higher in provinces with larger shares of Whites (ρ = .13), Coloured (ρ = .32), and other races (ρ = .12). A negligibly positive association was found between the provinces’ level of social cohesion and their share of Indian/Asian residents (ρ = .09). As to immigration, provinces with more immigrants were found to have a slightly stronger level of cohesion (ρ = .21).
The fractionalization measures offer findings that generally follow the tendencies mentioned above. Ethnic (racial) fractionalization exhibited a positive, though only weak, correlation with social cohesion (ρ = .16). The relationship with linguistic fractionalization emerged as positive and moderate (ρ = .43), whereas that with religious fractionalization was found to be weak and negative (ρ = -.12). Racial and linguistic diversity in the provinces seem to contribute to social cohesion, whereas religious diversity appears to harm it.
Modernization
The evidence is puzzling concerning educational attainment. Whereas the share of citizens with completed primary education exhibited a weakly positive correlation with social cohesion (ρ = .20), the shares of citizens with completed secondary education exhibited a negative, very strong and marginally significant correlation (ρ = -.67, p ≤ .10). Its size is comparable to the correlations of the social cohesion index with income inequality (P90/P10 ratio) and the share of singles. The correlation with the share of citizens with completed post-school education also emerged negative, though only weak (ρ = -.16). Provinces with better and more highly educated citizens seem to have lower levels of cohesion. A possible explanation for these surprising results could be the economy of the country which does not deliver jobs up to the expectations of the better educated citizens.
Access to modern information and communication technology (computers, cell phones, and the internet) poses yet another puzzle. The correlation of the social cohesion index is weakly to negligibly negative with the share of computer owners (ρ = -.10) and cell phone owners (ρ = -.10), but strongly positive with the share of the population without access to the internet (ρ = .62).
What brings social cohesion in the South African provinces forward is people-centered economic progress, rural population, marriages, racial and linguistic diversity, and immigration. As potential hazards to social cohesion emerged: unemployment, felt poverty, income inequality, high population density and urbanization, single life, older population, religious diversity, and access to the internet.
[1] In line with the customary practice in economic research and our previous studies, we transform the raw values by taking their natural logarithm (ln).
[2] Individuals below the food poverty line cannot afford enough food to obtain the minimum daily energy requirement for adequate health. Individuals below the lower-bound poverty line are unable to afford both adequate food and non-food items and have to sacrifice food for essential non-food items. Individuals below the upper-bound poverty line can afford adequate food and essential non-food items. In 2022, the food poverty line was at 663 Rand, the lower-bound poverty line at 945 Rand, and the upper-bound poverty line at 1417 Rand, according to the report of the Center for Risk Analysis (CRA, 2023).
[3] The Gini index measures income inequality in the population as a whole. It ranges from 0 (perfect equality among all individuals) to 1 (perfect inequality, where one individual has all income). The P90/P10 ratio contrasts the income at the 90th percentile of the income distribution to the income at its 10th percentile (OECD, 2021).
[4] Fractionalization is the probability that two randomly selected individuals are not from the same group (ethnic, linguistic, religious, etc.; Alesina et al., 2003). The corresponding indices for ethnic/linguistic/religious fractionalization range from 0 (all individuals are from the same ethnic group/speak the same language/are from the same religious group) to 1 (each individual belongs to a separate ethnic/linguistic/religious group).
5. Individual experiences of social cohesion
The previous sections of the report examined the levels and trends of social cohesion in South Africa and its provinces. Correlational analyses on the level of the provinces offered insights into potential structural characteristics that determine the local level of cohesion. In this section, we go down to the individual level to explore which population groups might be at risk of experiencing low cohesion in South Africa.
5.1 Data and method
There are several methodological approaches for identifying groups at risk of experiencing low cohesion, each involving different assumptions. One possibility is to perform separate analyses relating the individual scores on the overall cohesion index and its nine dimensions to the respondents' relevant socio-demographic and economic characteristics. This approach will likely lead to a plethora of results that are difficult to systematize. In order to reduce the complexity without a substantial loss of information, we prefer to identify classes (distinct groups) of respondents based on the pattern of their scores on the nine dimensions of social cohesion. The resulting classes are characterized by similarities within and dissimilarities across the classes concerning the experience of the nine aspects of cohesion by the respondents who belong to them. In a second step, we relate class membership to socio-demographic and socio-economic characteristics. In simpler terms, we investigate how the experience of social cohesion is related to individual characteristics. An example could be rich and poor citizens experiencing different levels of social cohesion.
To classify respondents into groups with distinct experiences of cohesion, we employ Latent Class Analysis. To cite from the abstract of a recent overview paper (Weller et al., 2020): “Latent class analysis (LCA) is a statistical procedure used to identify qualitatively different subgroups within populations who often share certain outward characteristics. The assumption underlying LCA is that membership in unobserved groups (or classes) can be explained by patterns of scores across survey questions, assessment indicators, or scales.” We take respondents’ scores on the nine dimensions of social cohesion in 2024 as the basis for the LCAs performed here.
LCAs are typically undertaken sequentially. The statistical procedure is programmed to come up with different numbers of groups, usually starting with two distinct groups (classes) and continuing until several groups (classes) are distinguished that offer plausible pathways of interpretation: Who are the people grouped into Class 1, Class 2, …, Class k? It is customary to summarize interpretations by labeling the different classes in a way that best characterizes their response patterns. Next to interpretability, specific indices of goodness-of-fit aid the decision of how many groups are most plausible to extract from the available data (Weller et al., 2020). These include the Akaike Information Criterion (AIC) and the (sample-size-adjusted) Bayesian Information Criterion (BIC, saBIC), which do not have pre-defined thresholds but inform comparisons of solutions: The solution with the lower AIC and (sa)BIC would be deemed better. Goodness-of-fit indices with pre-defined thresholds include the Entropy coefficient H and the Average Probability of Class Membership (APCM): Each should be greater than .90 for excellent fit or .80 for acceptable fit. Table A.21 of Appendix D documents the goodness-of-fit indices of five different LCA solutions. Table A.22 of Appendix D shows the population-weighted relative sizes of the classes in the total sample (N = 3172) from the various LCA solutions.
Based on the resulting goodness-of-fit indices of the LCA models we specified and considering the classes' interpretability, we selected the LCA model producing four classes.
5.2 Four classes of experience
Table 5.1 provides an overview of respondents’ average scores on the nine dimensions of cohesion, which served as the basis for the LCA, and the overall index of social cohesion within each class. Class 1 encompasses 18.6 % of the respondents. It is characterized by low cohesion with an average score of 37.5 out of 100 points. Classes 2 and 3 encompass 29.1 % and 41.8 % of the respondents, respectively. Both exhibit a moderate overall level of social cohesion with an average score of 54.6 in Class 2 and 54.4 in Class 3. Class 4, encompassing only 10.5 % of the respondents, exhibits a high level of social cohesion with an average score on the overall index of 73.3 points.
Table 1.8 Social cohesion and its dimensions in the four classes
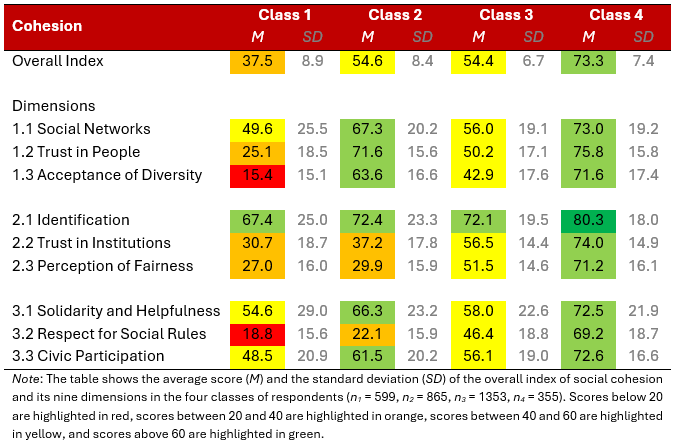
A closer look at the average scores of the classes on the nine dimensions reveals the aspects of cohesion along which the classes differ from each other (see Table 5.1 and Figure 5.1). Likely due to the large sample sizes of most classes, a series of Scheffe pairwise comparisons from one-way analyses of variance for each of the dimensions informs of significant differences (p ≤ 0.01) among all classes on all dimensions except for Identification between Class 2 (M = 72.4) and Class 3 (M = 72.1).
Respondents within Class 1 (low cohesion) exhibit a high level of identification (67.4). The strength of their social networks (49.6), their solidarity with weak others (54.6), and their involvement in civic life (48.5) is moderate. Members of Class 1 place low trust in others (25.1) and in institutions (30.7), and have a low perception of fairness (27.0). Their tolerance for diversity is very low (15.4). These respondents also have a very low perception that social rules are respected (18.8). Class 1 can be described as Critics.
Members of Class 2 (moderate cohesion) exhibit a mixed profile. They are well-networked socially (67.3), place high trust in others (71.6), and have high levels of tolerance for diversity (63.6), strong identification (72.4) as well as strong solidarity with weak others (66.3). Their involvement in civic life is also high (61.5). However, the trust they place in institutions is low (37.2), and they perceive low levels of fairness (29.9) and respect for rules (22.1). Class 2 can be described as Integrated sceptics.
Figure 1.6 Average scores of dimensions in the four classes
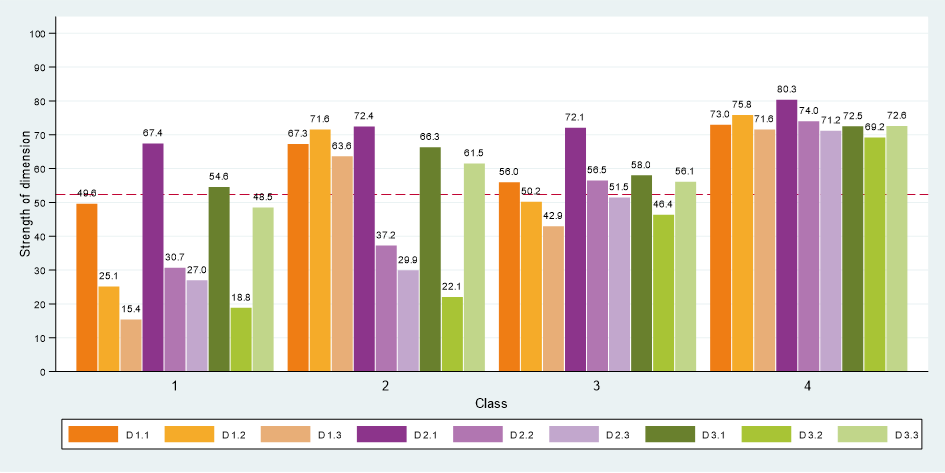
Note: The figure visualizes the average scores of the social cohesion dimensions in each of the four classes of respondents.
Members of Class 3 (moderate cohesion) rate most social cohesion aspects on the middle level. The strength of their solidarity with others (58.0) is moderate to high. Moderate are the level of trust they place in institutions (56.5), their involvement in civic life (56.1), the strength of their social networks (56.0), their level of perceived fairness (51.5), and the trust they place in other people (50.2). Further, these respondents have a moderate perception that rules are observed (46.4). Their tolerance for diversity, however, is moderate to low (42.9). Identification with the country (72.1) is the only strong aspect of cohesion in this class of respondents. If these were findings on the US society, we would label this class Middle America, with strong loyalty to the country and below-average acceptance of otherness. Class 3 can be described as Middle South Africa.
Members of Class 4 express a very strong identification with the country (80.3) and high levels of social cohesion in all aspects. Class 4 appears to be South Africa’s ideal-typical model of strong cohesion. It can be described as Cohesive communities.
Across all four classes, Identification with the country was found to be consistently high. In contrast, the deficits in Respect for social rules, particularly in Class 1 and Class 2, emerge yet again as an antithesis to citizens’ loyalty to the country.
5.3 Socio-demographics of the four classes
In this section, we explore which individual characteristics are typical for the four classes with distinct experiences of social cohesion. We do so using the following socio-demographic and socio-economic characteristics: biological sex (male, female), age group (18 to 24, 25 to 44, 45 to 64, 65 years and above), race (White, Black, Indian/Asian, Other), primary language (English, Afrikaans, Indigenous), marital status (single, married or living as married, windowed or divorced), community size (rural or village, town or city, metropolitan area), education (post-secondary, secondary, lower or none), employment status (employed, not in workforce, unemployed), and income class[1] (low, lower-middle, upper-middle, high, missing). Data on these characteristics stem from Wave 1 of Khayabus 2024.
The four classes were characterized in the framework of separate chi-square tests of independence between respondents’ class membership and the respective individual characteristics of interest. Table 5.2 documents the population-weighted relative frequencies (%) of the socio-demographic and socio-economic categories in the total sample and in each of the four classes as well as the respective result from the chi-square test of independence and Cramer’s V coefficient of effect size. Due to the large sample sizes in three of the classes, six out of nine tests emerged as statistically significant, but all effect sizes are small. Insignificant are the relationships between class membership and biological sex, marital status, and level of education. We, therefore, focus rather on the tendencies in the data.
Class 1, the Critics, is characterized by an overproportional representation of women (54.9 %), respondents from the age group 25-44 years (53.1 %), persons of other race (11.4 %), speakers of Afrikaans (17.0 %), singles (60.5 %), dwellers in towns or cities (30.0 %), unemployed (39.3 %), members of the low-income class (23.2 %) and persons who refused to report their household income (43.6 %). Class 1 is further characterized by an underrepresentation of men (45.1 %), respondents from the age group 45-64 years (24.1 %), Blacks (81.3 %), speakers of English (7.6 %), married or living together as married (29.9 %), dwellers in villages or rural areas (28.9 %), employed (40.2 %), and members of the lower-middle (9.4 %), upper-middle (13.2 %), and high-income class (10.6 %).
Class 2, the Integrated sceptics, is characterized by an overproportional representation of Whites (10.4 %) and Indian/Asian (4.1 %), speakers of English (14 %) and Afrikaans (16.7 %), respondents who have completed secondary (50.6 %) and post-secondary education (17.3 %), employed (47.7 %), and members of the high-income class (19.1 %). Conversely, Class 2 is characterized by an underproportional representation of respondents from the age group 18-24 years (13 %), Blacks (76.8 %), speakers of indigenous languages (69.3 %), respondents who have low or no completed education (32.1 %), respondents who are not in the workforce (15.8 %), and members of the low-income class (18.2 %).
Class 3, Middle South Africa, is characterized by an overproportional representation of respondents from the age group 18-24 years (17.2 %), Blacks (87.7 %), speakers of indigenous languages (79.7 %), dwellers in towns and cities (29.0 %), respondents with low or no completed education (38.4 %), respondents who are not in the workforce (21.9 %), and members of the low (25.0 %) and lower-middle income class (12.9 %). In contrast, Class 3 is characterized by an underproportional representation of respondents from the age group 25-44 years (48.7 %), non-Blacks (Whites – 4.3 %, Indian/Asian – 1.4 %, Other – 6.6 %), speakers of Afrikaans (10.3 %), dwellers in metropolitan areas (40.0 %), respondents who have completed secondary education (47.5 %), employed (41.7 %), and respondents who have refused to report their household income (33.4 %).
Table 1.9 Socio-demographic and economic characteristics of the four classes of respondents
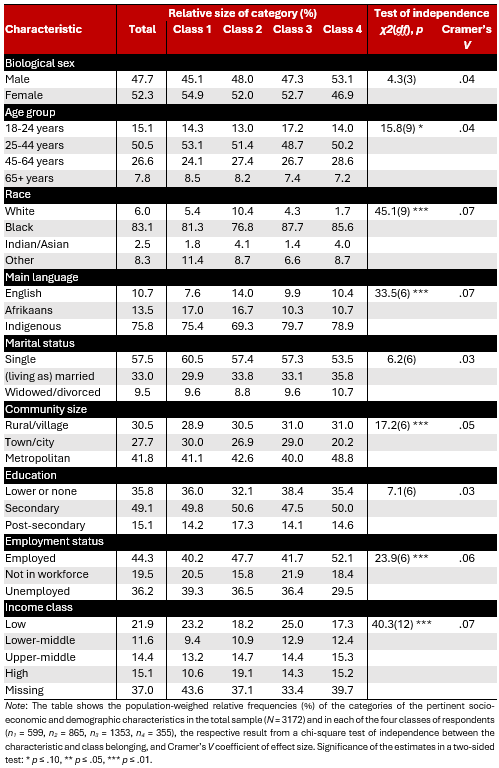
Class 4, the Cohesive Communities, is characterized by an overproportional representation of men (53.1 %), respondents from the age group 45-64 years (28.6 %), Blacks (85.6 %) and Indian/Asian (4.0 %), speakers of indigenous languages (78.9 %), married or living together as married (35.8 %), divorced or widowed (10.7 %), dwellers in metropolitan areas (48.8 %), employed (52.1 %), and respondents who have refused to report their household income (39.7 %). Conversely, Class 4 is characterized by an underrepresentation of women (46.9 %), respondents from the age group 18-24 years (14.0 %), Whites (1.7 %), speakers of Afrikaans (10.7 %), singles (53.5 %), dwellers in towns or cities (20.2 %), respondents who are not in the workforce (18.4 %), unemployed (29.5 %), and members of the low-income class (17.3 %).
[1] Income classes were derived from the reported total household income. The latter was equivalized concerning household size using the modified OECD equivalence scale. Respondents with equivalized household income lower than 60 % of the median belong to the low-income class, from 60 % to 100 % - to the lower-middle income class, from 100 % to 200 % - to the upper-middle income class, greater than 200 % - to the high-income class. Respondents with a missing value on household income are treated as a separate group due to the large share of non-response (41.5 %).
6. Social cohesion and subjective well-being
In the present section, we inspect the data to determine whether they support the consistent finding from our previous studies on the topic that high levels of social cohesion are related to greater (subjective) well-being. We employ the following five items from the Khayabus survey as proxies of well-being:
Think of the way your family lives, would you say that your family is… better off than a year ago / about the same / worse off than a year ago?
And how do you think your family’s lives will be in a year’s time? Do you think your family will be… better off than today / about the same / worse off than today?
Please think about your children or the children of family or friends. What do you think the future holds for these children? Do you think that… they have a bright future ahead of them / they have a bleak future ahead of them?
And your satisfaction with life? Has it… improved/staying the same/worse compared to a few months ago?
On a scale from 1 to 5 please indicate whether you (1) strongly disagree, (2) disagree, (3) neither agree nor disagree, (4) agree or (5) strongly agree with the following statement: I am seriously considering emigrating to another country in the next year or so.
We perform analyses both on the level of provinces and of individuals.
6.1 Provinces
We aggregate the individual responses to the five items to measure well-being on the level of provinces. For each province, we take the respective share of the positive response option to each of the four items with categorically scaled answers (better off, bright future, improved) and the arithmetic mean of the individual responses to the Likert-scale item tapping on emigration.
We apply the same methodological approach as in Section 4, which explored associations between several structural characteristics of the provinces and the index of social cohesion. Table A.20 of Appendix C documents the biserial bivariate correlations on the individual level and the bivariate and partial Pearson correlations on the level of provinces. As in Section 4, we report and interpret the results from the Spearman correlations partialled for GDP (see Table 6.1).
Table 1.10 Social cohesion and subjective well-being in the provinces
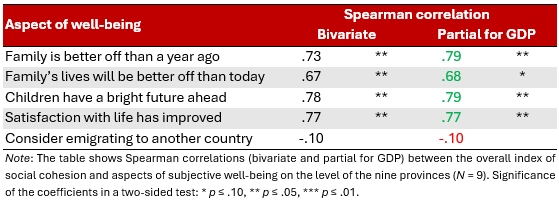
The share of respondents evaluating their family’s life as better off today than a year ago exhibits a very strong positive correlation with the level of social cohesion in respondents’ province (ρ = .79). The same was found for the share of respondents evaluating their life satisfaction as improved in comparison to a few months ago (ρ = .77). Further, in more cohesive provinces, there are larger shares of respondents believing that their family’s lives will be better off than today in a year (ρ = .68) and that their children will have a bright future ahead (ρ = .79). The share of respondents who consider emigrating to another country tends to be negligibly lower in the more cohesive provinces (ρ = -.10).
The provinces' results indicate that social cohesion is conducive to a positive life evaluation, higher life satisfaction, and greater optimism. They are entirely in line with our findings from other continents: The higher the level of social cohesion in a geopolitical entity, the more positive people’s outlook on life (see Dragolov et al., 2016; Bertelsmann Stiftung, 2018; Arant et al., 2017; Boehnke et al., 2024).
6.2 Individuals
To investigate the association between social cohesion and subjective well-being on the individual level, we relate the individual responses to the well-being items to respondents’ membership in the four distinct classes of experiencing social cohesion. As most of the items on well-being are of categorical measurement quality, we apply the approach from Section 5 to describe the four classes based on respondents’ socio-demographic and socio-economic characteristics. Table 6.2 documents the results. Unlike the very weak relationships found in Section 5, the individual experience of social cohesion exhibits somewhat stronger associations with four of the indicators of subjective well-being.
Table 1.11 Subjective well-being in the four classes of respondents
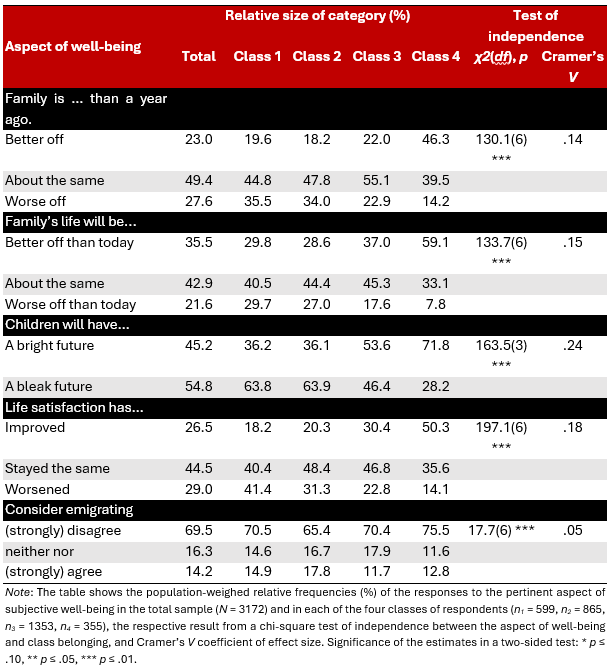
The evidence presents a clear picture. The greatest share of respondents who evaluate their family’s life as better off than a year ago was found in Class 4, Cohesive Communities (46.3 %). The second largest share was found in Class 3, Middle South Africa (22 %). The lowest shares were found in Class 2, Integrated sceptics (18.2 %), and Class 1, Critics (19.6 %). The largest shares of respondents evaluating their family’s life as worse off than a year ago were found in Class 1 (35.5 %) and Class 2 (34 %).
Moving on to the two indicators of optimism, we find similar evidence. The largest share of respondents believing their family’s life would be better off than today in a year was found again in Class 4 (59.1 %). Class 3 appears somewhat reserved, given that most believed life would be about the same (45.3 %). Again, in Class 1 (29.7 %) and Class 2 (27 %), we find the largest shares of respondents who believe their family’s life would be worse off than today. It should be mentioned, though, that within Class 1 and Class 2, the share of pessimists is counterbalanced by an approximately similarly sized share of optimists. Optimism regarding children’s future was found highest in Class 4, followed by Class 3: 71.8 % and 53.6 %, respectively, believe children would have a bright future ahead of them. Pessimism prevails in the other two classes, where about 64 % of the respondents believe children would have a bleak future ahead of them.
The above-described pattern holds for life satisfaction, too. The largest share of respondents evaluating their life satisfaction as improved was found in Class 4 (50.3 %), followed by Class 3 (30.4 %). Life satisfaction has worsened for the majority in Class 1 (41.4 %). Most of the respondents in Class 2 (48.4 %) and Class 3 (46.8 %) reported that their life satisfaction has stayed the same. And yet, in Class 3, the share of respondents for whom life satisfaction has increased (30.4 %) is larger than the share of those for whom it has worsened (22.8 %); this pattern is reversed in Class 2.
Finally, the association between the experience of social cohesion and emigration is rather blurred. An overwhelming majority in each class (65.4% in Class 2 and over 70 % in the other classes) disagreed or strongly disagreed with the statement, “I am seriously considering emigrating to another country in the next year or so.” The disagreement rate is highest in Class 4 (75.5 %). Class 2 has the relatively highest rate of agreement with the statement (17.8 %), followed by Class 1 (14.9 %) and Class 4 (12.8 %), whereas only 11.7 % of Class 3 consider emigrating. High social cohesion appears insufficient to discourage people from emigrating. The relationship is, however, very weak in terms of effect size.
7. Discussion and conclusion
South Africa has debated social cohesion ever since the end of the apartheid regime, especially in light of the Rainbow Nation concept. However, only two empirical studies have assessed South Africa's social cohesion during all these years. The insights that this past research provided are, by and large, piecemeal and outdated by now.
The present study aimed to assess social cohesion in South Africa, tracking its development from 2021 to 2024; to identify structural factors that promote or impede it; to explore social groups at risk of experiencing low cohesion, and to examine the relationship between social cohesion and citizens' well-being. Cohesion was conceptualized and operationalized along the measurement concept of the Bertelsmann Social Cohesion Radar which has been used as a model to quantify societal cohesiveness in altogether over 60 countries across the world. The study drew on the data from Waves 1 of the Khayabus survey, a population-representative cross-sectional survey fielded annually by IPSOS South Africa. Below, we summarize and discuss the results in light of previous evidence but mostly leave the conclusions to the political bodies engaging with the study results.
In 2024, the overall level of social cohesion in South Africa as a whole was found moderate at 53.3 points (of maximum 100). It went through a steady, though slow, decline from 53.5 points in 2021, the starting point of our analysis, to 51.7 points in 2023. In 2023, the downward trend in South Africa’s overall level of social cohesion halted and reversed in an upward direction. The previously accrued decline has been almost overcome. Yet, one needs to consider that our study offers insights on the recent four years only of altogether three decades since apartheid – a new chapter in South Africa’s history whose onset was marked by hope, reconciliation and the vision to create a Rainbow Nation. The current – only moderate – level of cohesion suggests that the South African society is perhaps still far from its ambitious founding goal. The glue that holds the South African society together consists mostly of the high level of identification with the country (72.2) but also of high solidarity with weaker fellow citizens (61.3) and relatively well functioning social networks (59.9). Political attention must, however, be paid to the widely perceived lack of respect for social rules (36.6), as it can spark turmoil. Policy makers may also consider overcoming the perceived lack of distributional fairness (42.7), strengthening acceptance of diversity (46.8) and trust in institutions (47.9).
To put the findings on South Africa in a comparative perspective, we refer to the case of Germany (see Boehnke et al., 2024). Interestingly, Germany’s level of social cohesion in 2023 (52 points) was almost identical to South Africa’s in the same year. Germany also experienced a downward, though much steeper, trend, losing nine points on the overall index between 2020 and 2023. The COVID pandemic is often seen as one of the reasons for dwindling social cohesion. In the authors’ view, which for reasons of data availability is not backed by empirical data, a reversal in the downward trend in Germany is not in sight yet, considering the intensifying societal and political polarization on pressing issues such as the inflation, the Ukraine-Russia military conflict, migration and violent crime, to name a few. A comparison of the results from 2023 further reveals that the two countries do not have identical profiles of cohesion. What they have in common are a similar strength of Social networks, practically the same level of Trust in institutions, and a lacking Perception of fairness. Germany’s profile is much stronger with respect to Acceptance of diversity and, quite stereotypically, Respect for social rules, but weaker with respect to Trust in people, Identification, Civic participation, and particularly Solidarity and helpfulness (34 points). Extending the scope to the comparison of 34 EU and OECD member states (Dragolov et al., 2016), we find considerable similarities in the pattern of strengths and weakness between South Africa and Israel. The society of Israel exhibits similarly strong Identification, moderate Solidarity, Social networks, and Trust in people, as well as pronounced deficits in the Perception of fairness and Respect for social rules. No country among the 22 Asian studied (Bertelsmann Stiftung, 2018) exhibits this profile. Nevertheless, in light of South Africa’s membership in BRICS, we briefly outline the cohesion profiles of India and China for comparison purposes. India’s profile is characterized by very weak Social networks, low Trust in people, very low Acceptance of diversity, very low Identification, moderate Trust in institutions, very low Perception of fairness, low Solidarity and helpfulness, moderate Respect for social rules, and moderate Civic participation. In contrast, China’s profile is characterized by strong Social networks, very high Trust in people, moderate Acceptance of diversity, moderate Identification, very high Trust in institutions, moderate Perception of fairness, very low Solidarity and helpfulness, high Respect for social rules, and very low Civic participation.
The survey data further allowed us to examine cohesion in the nine South African provinces. In 2024 as well as in all previous years analyzed, all provinces achieved scores on the overall index in the interval designating a moderate level of cohesion. Yet, despite an improvement of +3.5 points from 2023 to 2024, KwaZulu-Natal had (again) the relatively lowest level of social cohesion (49.6). Below the South African average are currently also the provinces of North West (52.0), Free State (52.1), and Mpumalanga (53.2). Limpopo (56.5) had (again) the relatively highest level of social cohesion than the rest of the country, despite a decline of -1.8 points from 2023 to 2024.
The look into people’s subjective experience of social cohesion yielded four groups (classes) with distinctive patterns of strengths and weakness. Class 4, Cohesive communities, emerged in 2024 as an ideal-typical model of strong cohesion but represents only some 10 % of the South African society. In contrast, Class 1, the Critics, did emerge also in 2024 as the segment of the population (18.6 %) that experiences worrying deficits in five of altogether nine aspects of cohesion, most alarming of which are the critically low levels of acceptance of diversity (15.4 points) and respect for social rules (18.8 points). This at-risk population segment encompasses overproportionally more women, respondents from the age group 25-44 years, persons of other race, speakers of Afrikaans, singles, dwellers in towns or cities, unemployed, and members of the low-income class. It would be an overstatement and, moreover, methodologically inappropriate, to conclude that, i.e., all women or all low-income earners are at risk of experiencing low social cohesion. However, some of the above listed social groups and, in particular, combinations thereof, certainly deserve policy makers’ attention.
Next, several surprising results on the associations between the structural characteristics of the provinces and their level of social cohesion need to be highlighted. Previous studies elsewhere have always yielded that geopolitical entities enjoying higher levels of per capita GDP also exhibit higher social cohesion, Scandinavia being the most prominent example. Findings for Asian countries corroborate that result (Delhey et al., 2018). In South Africa, the wealth of the provinces is unrelated to their level of cohesion. Our study, furthermore, finds conflicting results on poverty and inequality. On the one hand, social cohesion is lower in provinces where more households view themselves as poor but not lower in provinces where more people objectively live in poverty. That poverty harms cohesion has been a consistent finding in our studies on Western societies (Dragolov et al., 2016), Asian societies (Bertelsmann Stiftung, 2018) as well as Germany’s federal states and regions (Arant et al., 2017; Boehnke at al., 2024). On the other hand, though, social cohesion is lower in provinces with larger discrepancies in the income distribution, which is in line with the results on Western countries. Further, a negative link with median age (the younger the people in an area, the higher the level of social cohesion) was discovered, which appears counterintuitive at first glance. Another somewhat puzzling result is the positive correlation with ethnic and linguistic fractionalization. However, evidence on Asian societies shows that ethnic and linguistic diversity are conducive to social cohesion up to a certain extent and harmful beyond it. Another counterintuitive result is the positive association between the level of social cohesion in the provinces and the share of citizens without access to the internet. By no means should the latter finding be interpreted such that wider provision of access to the internet per se harms social cohesion. The internet is merely a tool. It empowers people by facilitating, e.g., economic activity, access to knowledge, and social interactions, but also has its dark sides promoting criminal activity, the spread of fake news and misinformation as well as discursive polarization. Further research is needed to shed more light on the mechanism operating behind the finding at stake, but one thing is for sure: One should not throw the baby out with the dirty bathwater.
Beside the counterintuitive and surprising results discussed above, the analyses of the provinces’ structural characteristics offer insights on how to strengthen social cohesion. To begin with, the provinces’ level of social cohesion was found to correlate positively with the Human Development Index, and negatively with subjective poverty, unemployment, and income inequality. These findings suggest that social cohesion can be enhanced by enabling inclusive (people-centered) economic progress, reducing unemployment, and lowering income inequality. In addition, we found negative associations between the provinces’ level of social cohesion and the shares of citizens with completed secondary and post-secondary degrees. We assume that this is due to an underperformance of the South African economy in providing adequate jobs for the highly qualified. Next, cohesion in the provinces was found to correlate positively with the share of married citizens – conversely, negatively with the share of singles. The latter finding suggests that social cohesion can be fostered by promoting a family-oriented life-style. Finally, we found a positive relationship between the provinces’ level of social cohesion and the share of rural population – conversely, a negative relationship with the share of urban population. It is likely that dwellers in rural areas feel forced to leave their otherwise supportive rural communities in a pursuit of better living conditions and greater economic opportunities in the urban centers, where social cohesion tends to be lower. This could be avoided by efforts to bring the living conditions in rural and urban areas to an equally adequate level.
And yet, what is social cohesion good for? The present study on South Africa corroborates a core finding of essentially all other studies we have performed to measure social cohesion elsewhere: The quality of society (social cohesion) translates directly into citizens’ quality of life (subjective well-being). In more technical terms: The higher social cohesion is, the better and more satisfying life people have, and the more optimistic they are about the future.
References
Abrahams, C. (2016). Twenty years of social cohesion and nation-building in South Africa. Journal of Southern African Studies, 42(1), 95–107. http://www.jstor.org/stable/43900558
Alesina, A., Devleeschauwer, A., Easterly, W., Kurlat, S., & Wacziarg, R. (2003). Fractionalization. Journal of Economic Growth 8, 155–194. https://doi.org/10.1023/A:1024471506938
Apraku, A., Moyo, P., & Akpan, W. (2018). Coping with climate change in Africa: an analysis of local interpretations in Eastern Cape, SA. Development Southern Africa, 36(3), 295–308. https://doi.org/10.1080/0376835X.2018.1482199
Arant, R., Dragolov, G., & Boehnke, K. (2017). Sozialer Zusammenhalt in Deutschland 2017. Gütersloh: Bertelsmann Stiftung.
Arant, R., Larsen, M., & Boehnke, K. (2016). Sozialer Zusammenhalt in Bremen. Gütersloh: Bertelsmann Stiftung.
Ballantine, C., Chapman, M., Erwin, K., & Maré, G. (Eds.) (2017). Living together, living apart? Social cohesion in a future South Africa. University of Kwa-Zulu Natal Press. http://www.ukznpress.co.za/?class=bb_ukzn_books&method=view_books&global%5Bfields%5D%5B_id%5D=496
Bertelsmann Stiftung (Ed.). (2018). What holds Asian societies together: Insights from the Social Cohesion Radar. Gütersloh: Bertelsmann-Stiftung.
Boehnke, K., Berrueto, A., Dragolov, G., & Ocampo Villegas, P. (2019). Are value preferences and social cohesion interconnected? The case of Mexico. Acta de Investigación, 9(2). http://dx.doi.org/10.22201/fpsi.20074719e.2019.2.262
Boehnke, K., Dragolov, G., Arant, R., & Unzicker, K. (2024). Gesellschaftlicher Zusammenhalt in Deutschland 2023. Gütersloh: Bertelsmann Stiftung.
Burns, J., Hull, G., Lefko-Everett K., & Njozela, L. (2018). Defining social cohesion (SALDRU Working Paper 216). Cape Town: SALDRU, UCT.
Center for Risk Analysis. (2023). Assets and Incomes. November 2023. Johannesburg: Center for Risk Analysis.
Delhey, J. & Boehnke, K. (2018). Conceptualizing social cohesion in Asia, In Bertelsmann Stiftung (Ed.), What holds Asian societies together: Insights from the Social Cohesion Radar (pp. 29-48). Gütersloh: Bertelsmann-Stiftung.
Delhey, J., Boehnke, K., Dragolov, G., Ignácz, Z.S., Larsen, M., Lorenz, J., & Koch, M. (2018). Social Cohesion and its Correlates: A Comparison of Western and Asian Societies. Comparative Sociology, 17(3-4), 426–455. https://doi.org/10.1163/15691330-12341468
Delhey, J., Dragolov, G. & Boehnke, K. (2023). Social Cohesion in International Comparison: A Review of Key Measures and Findings. Kölner Zeitschrift für Soziologie und Sozialpsychologie, 75, 95-120. https://doi.org/10.1007/s11577-023-00891-6
Dragolov, G., Ignácz, Z. S., Lorenz, J., Delhey, J., Boehnke, K., & Unzicker, K. (2016). Social cohesion in the Western world. What holds societies together: Insights from the Social Cohesion Radar. Springer International. https://doi.org/10.1007/978-3-319-32464-7
Global Data Lab (2024). Subnational HDI (v8.0). Retrieved on September 24, 2024 from https://globaldatalab.org/shdi/table/shdi/ZAF/
Langer, A., Stewart, F., Smedts, K., & Demarest, L. (2017). Conceptualising and measuring social cohe-sion in Africa: Towards a perceptions-based index. Social Indicators Research, 131, 321-343. https://doi.org/10.1007/s11205-016-1250-4
Larsen, M. M., & Boehnke, K. (2016). Measuring Social Cohesion in the Kyrgyz Republic. Social Cohesion Index. University of Central Asia's Institute of Public Policy and Administration Working Paper No. 36, Bishkek.
Leininger, J., Burchi, F., Fiedler, C., Mross, K., Nowack, D., von Schiller, A., Sommer, C., Strupat, C., & Ziaja, S. (2021). Social cohesion: A new definition and a proposal for its measurement in Africa (Discussion Paper 31/2021). Bonn: Deutsches Institut für Entwicklungspolitik (DIE). https://doi.org/10.23661/dp31.2021.v1.1
OECD (2021). Old-age income inequality. In Pensions at a Glance 2021: OECD and G20 Indicators. OECD Publishing, Paris. doi: https://doi.org/10.1787/d1a5a309-en
Schiefer, D. & van der Noll, J. (2017). The essentials of social cohesion: A literature review. Social Indicators Research, 132(2), 579-603. https://doi.org/10.1007/s11205-016-1314-5
Statistics South Africa (2023a). Census 2022. Pretoria: Stats SA.
Statistics South Africa (2023b). Quarterly Labour Force Survey. Quarter 4: 2022. Pretoria: Stats SA.
Statistics South Africa (2024a). Estimation of regional gross domestic product for South Africa: Experimental estimates. Pretoria: Stats SA.
Statistics South Africa (2024b). Population Estimates. Pretoria: Stats SA
Statistics South Africa (2024c). Subjective poverty in South Africa. Findings from the General Household Surveys 2019 and 2022. Pretoria: Stats SA.
Wasserstein, R. L., Schirm, A. L., & Lazar, N. A. (2019). Moving to a World Beyond “p < 0.05.” The American Statistician, 73(sup1), 1–19. https://doi.org/10.1080/00031305.2019.1583913
Weller, B. E., Bowen, N. K., & Faubert, S. J. (2020). Latent Class Analysis: A guide to best practice. Journal of Black Psychology, 46(4), 287-311. https://doi.org/10.1177/0095798420930932
Appendices
Appendix A: Indicators of cohesion across time
This Appendix documents the population-weighted relative frequencies of the response categories of the indicators used to calculate the social cohesion scores in the report. The reported values pertain to the respective percentage distributions in the total sample for each year of data collection.
Table A.12 Indicators of Dimension 1.1 “Social networks” across time
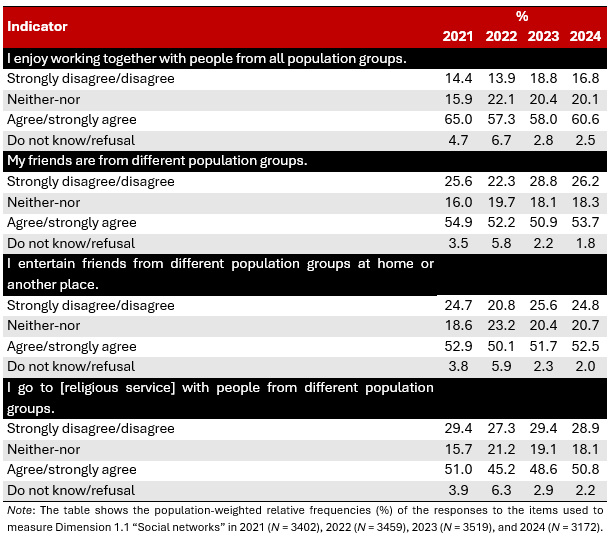
Table A.13 Indicators of Dimension 1.2 “Trust in people” across time
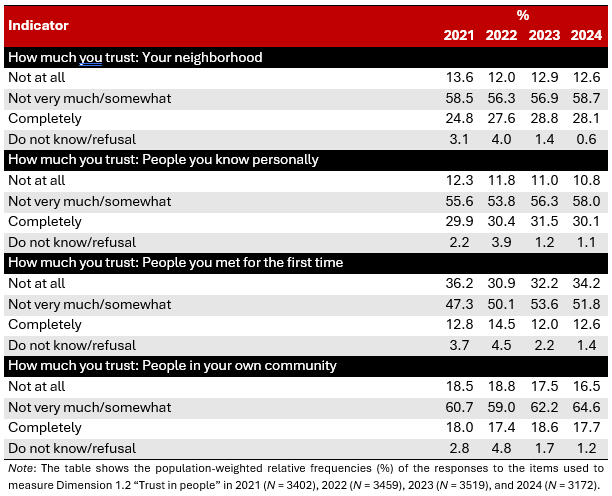
Table A.14 Indicators of Dimension 1.3 “Acceptance of diversity” across time
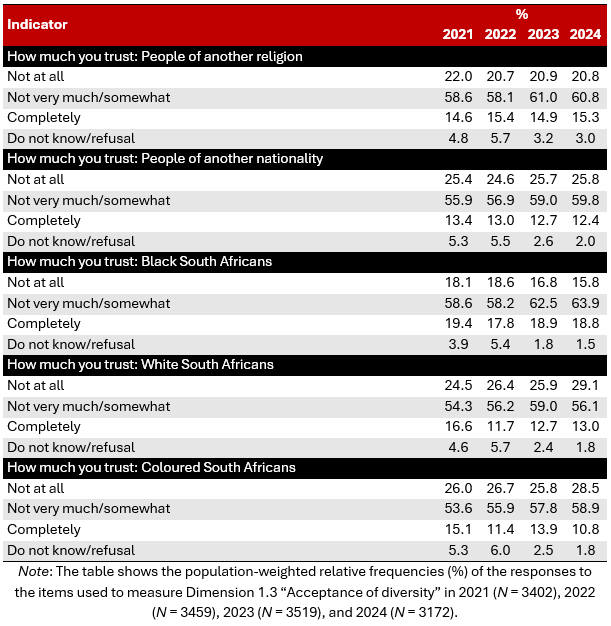
Table A.15 Indicators of Dimension 2.1 “Identification” across time
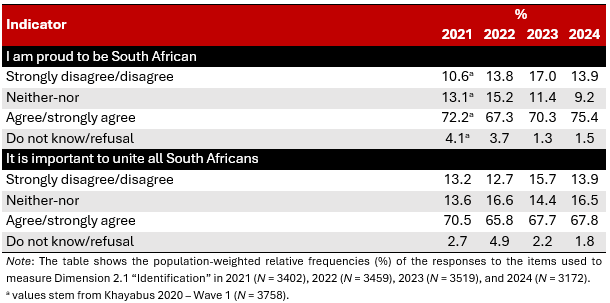
Table A.16 Indicators of Dimension 2.2 “Trust in institutions” across time
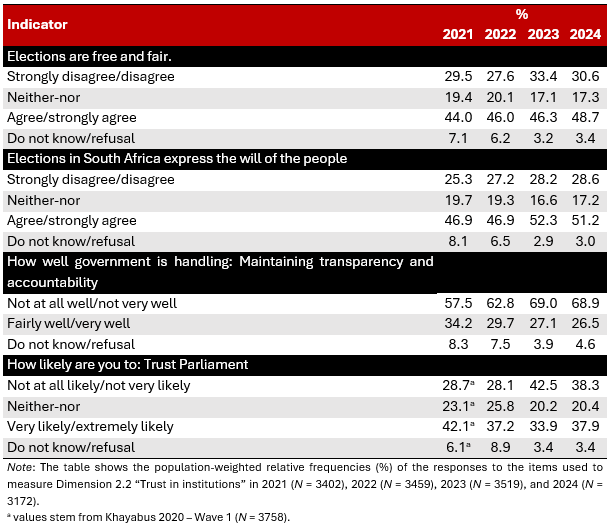
Table A.17 Indicators of Dimension 2.3 “Perception of fairness” across time

Table A.18 Indicators of Dimension 3.1 “Solidarity and helpfulness” across time
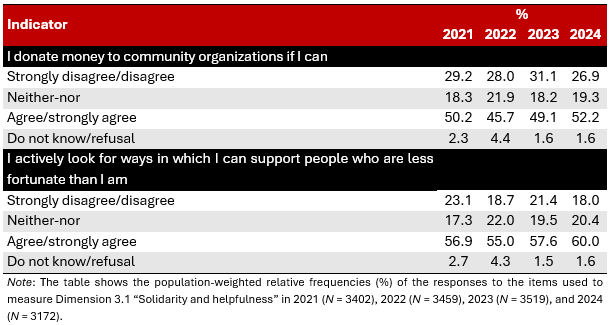
Table A.19 Indicators of Dimensions 3.2 “Respect for social rules” across time

Table A.20 Indicators of Dimension 3.3 “Civic participation” across time

Appendix B: Dimensions of cohesion in the provinces over time
This Appendix contains results on the level and change over time in the nine single dimensions of social cohesion across the provinces of South Africa.
Domain “Social Relations”
Table A.21 Dimension 1.1 “Social networks” in the provinces across time

Table A.22 Dimension 1.2 “Trust in people” in the provinces across time

Table A.23 Dimension 1.3 “Acceptance of diversity” in the provinces across time

Domain “Connectedness”
Table A.24 Dimension 2.1 “Identification” in the provinces across time
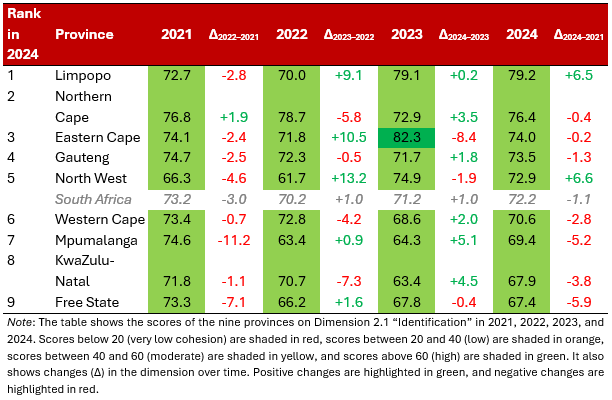
Table A.25 Dimension 2.2 “Trust in institutions” in the provinces across time
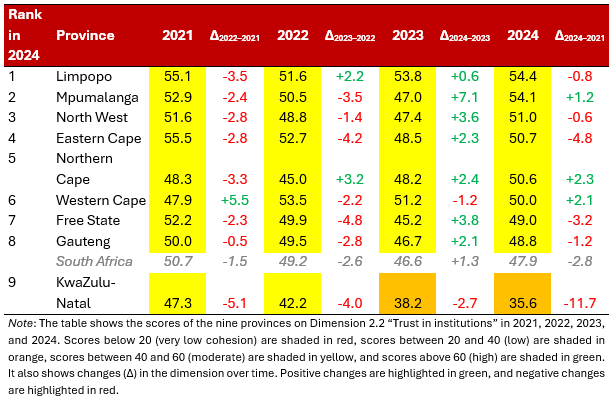
Table A.26 Dimension 2.3 “Perception of fairness” in the provinces across time
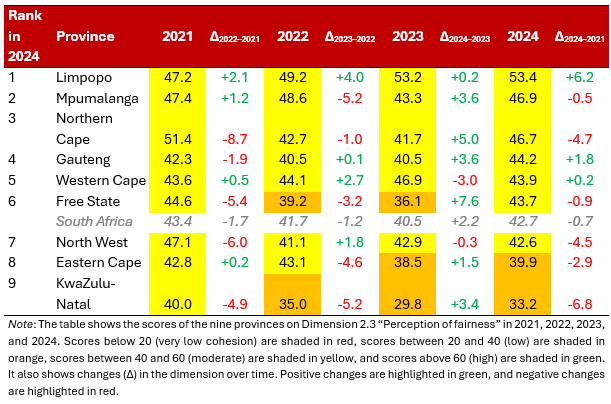
Domain “Focus on the Common Good”
Table A.27 Dimension 3.1 “Solidarity and helpfulness” in the provinces across time
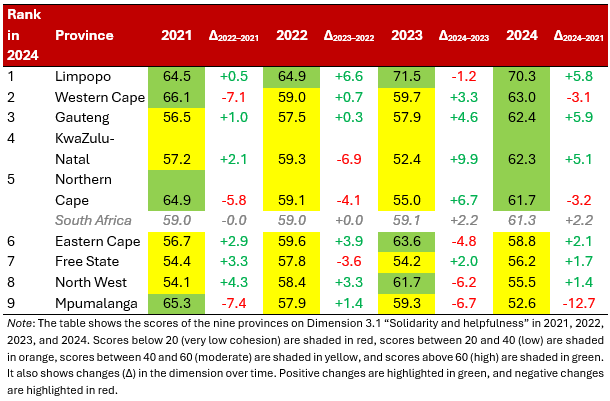
Table A.28 Dimension 3.2 “Respect for social rules” in the provinces across time
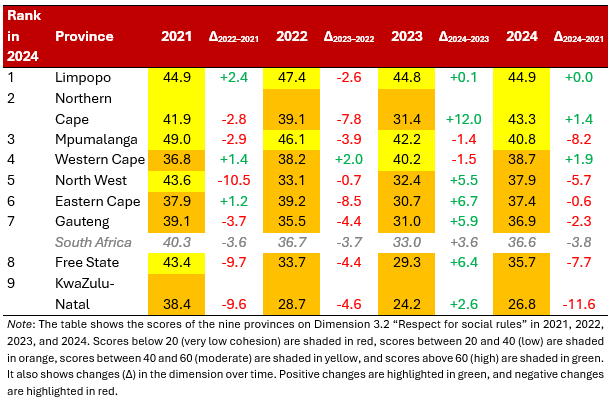
Table A.29 Dimension 3.3 “Civic participation“ in the provinces across time
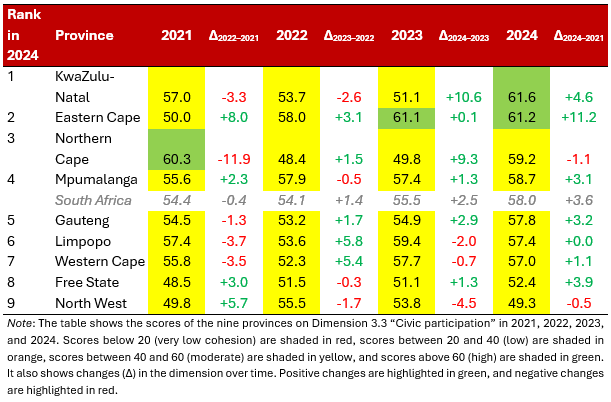
Appendix C: Correlations of social cohesion on the province level
Table A.30 Structural characteristics and social cohesion in South African provinces
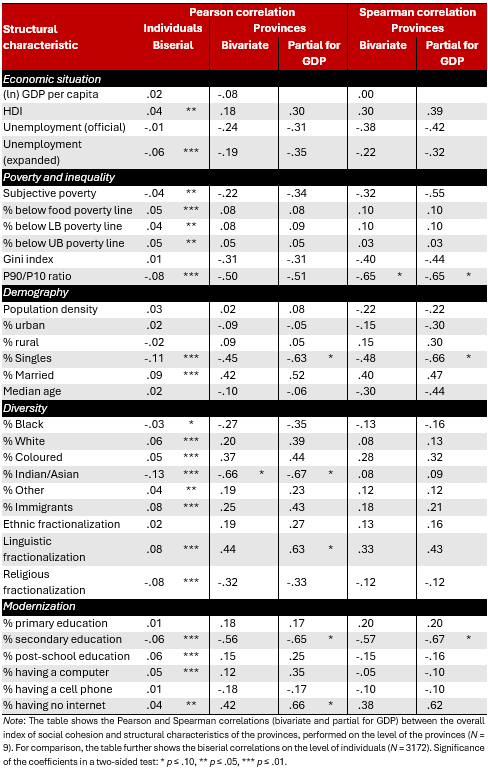
Table A.31 Social cohesion and subjective well-being in the provinces
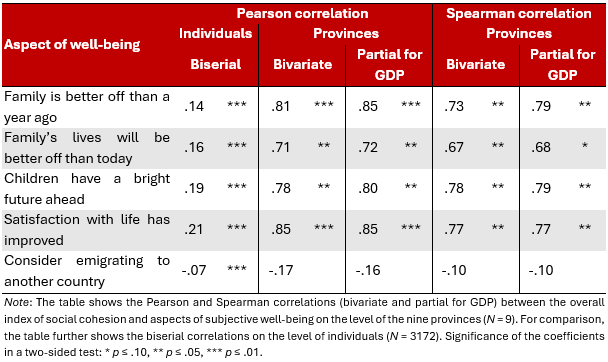
Appendix D: Latent class analyses
This Appendix documents goodness-of-fit indices for the various LCA models specified.
Table A.32 Goodness-of-fit indices of LCA solutions
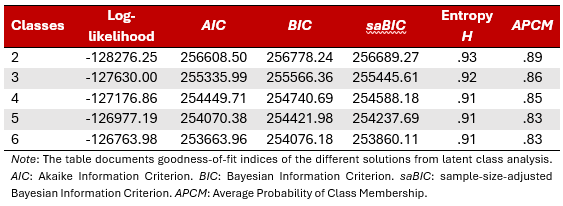
Table A.33 Relative class sizes for LCA solutions
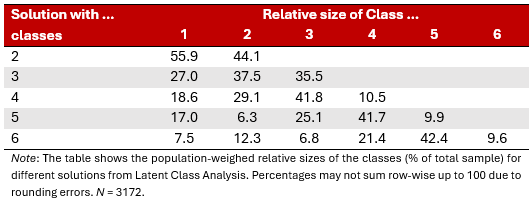
- - - - - - - - - - - - - - - - - - - - - - - - - - - - - - - - -
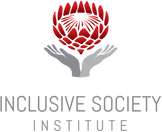
This report has been published by the Inclusive Society Institute
The Inclusive Society Institute (ISI) is an autonomous and independent institution that functions independently from any other entity. It is founded for the purpose of supporting and further deepening multi-party democracy. The ISI’s work is motivated by its desire to achieve non-racialism, non-sexism, social justice and cohesion, economic development and equality in South Africa, through a value system that embodies the social and national democratic principles associated with a developmental state. It recognises that a well-functioning democracy requires well-functioning political formations that are suitably equipped and capacitated. It further acknowledges that South Africa is inextricably linked to the ever transforming and interdependent global world, which necessitates international and multilateral cooperation. As such, the ISI also seeks to achieve its ideals at a global level through cooperation with like-minded parties and organs of civil society who share its basic values. In South Africa, ISI’s ideological positioning is aligned with that of the current ruling party and others in broader society with similar ideals.
Email: info@inclusivesociety.org.za
Phone: +27 (0) 21 201 1589
Comments